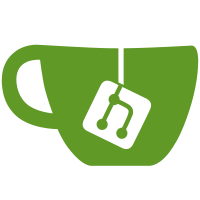
@ -1,60 +0,0 @@
|
||||
# Project01 - First Kaggle: Titanic - audit |
||||
|
||||
### Preliminary |
||||
|
||||
``` |
||||
project |
||||
│ README.md |
||||
│ environment.yml |
||||
│ username.txt |
||||
│ |
||||
└───data |
||||
│ │ train.csv |
||||
│ | test.csv |
||||
| | gender_submission.csv |
||||
│ |
||||
└───notebook |
||||
│ │ EDA.ipynb |
||||
| |
||||
|───scripts |
||||
│ |
||||
|
||||
``` |
||||
|
||||
###### Does the structure of the project is as below ? |
||||
|
||||
|
||||
###### Does the readme file give an introduction of the project, show the username, describe the feature engineering and show the best score the on the leaderboard ? |
||||
|
||||
###### Does the environment contain all libraries used and their versions that are necessary to run the code ? |
||||
|
||||
|
||||
|
||||
### Feature engineering |
||||
|
||||
|
||||
###### Does the notebook can be executed without any arror ? |
||||
|
||||
###### Does the notebook explain the feature engineering that contributed to improve the accuracy ? |
||||
|
||||
### Scripts |
||||
|
||||
###### Can you train the best model on the train data with feature engineering without any error ? |
||||
|
||||
###### Can you predict on the test set using the best model without any error ? |
||||
|
||||
###### Is the score you get **on the test set** with the best model is close to what is expected ? |
||||
|
||||
|
||||
### Final score |
||||
|
||||
###### Is the accuracy associated with the username in `username.txt` is higher than 79% ? The best submission score can be accessed from the user profile. |
||||
|
||||
|
||||
### Examples |
||||
|
||||
Here are two very good submissions explained and detailed: |
||||
|
||||
- https://www.kaggle.com/konstantinmasich/titanic-0-82-0-83 |
||||
- https://www.kaggle.com/sreevishnudamodaran/ultimate-eda-fe-neural-network-model-top-2 |
||||
|
@ -1,116 +0,0 @@
|
||||
# Your first Kaggle: Titanic |
||||
|
||||
## Introduction |
||||
|
||||
The goal of this **1 week** project is to get the highest possible score on a Data Science competition. More precisely you will have to predict who survived the Titanic crash. |
||||
|
||||
![alt text][titanic] |
||||
|
||||
[titanic]: titanic.jpg "Titanic" |
||||
|
||||
### Kaggle |
||||
|
||||
Kaggle is an online community of data scientists and machine learning practitioners. Kaggle allows users to find and publish data sets, explore and build models in a web-based data-science environment, work with other data scientists and machine learning engineers, and enter competitions to solve data science challenges. It’s a crowd-sourced platform to attract, nurture, train and challenge data scientists from all around the world to solve data science, machine learning and predictive analytics problems. |
||||
|
||||
### Titanic - Machine Learning from Disaster |
||||
|
||||
One of the first Kaggle competition I did was: Titanic - Machine Learning from Disaster. This is a not-to-be-missed Kaggle competition. |
||||
|
||||
You can see more [here](https://www.kaggle.com/c/titanic) |
||||
|
||||
The sinking of the Titanic is one of the most infamous shipwrecks in history. On April 15, 1912, during her maiden voyage, the widely considered “unsinkable” RMS Titanic sank after colliding with an iceberg. Unfortunately, there were not enough lifeboats for everyone onboard, resulting in the death of 1502 out of 2224 passengers and crew. |
||||
|
||||
While there was some element of luck involved in surviving, it seems some groups of people were more likely to survive than others. |
||||
|
||||
|
||||
In this challenge, you have to build a predictive model that answers the question: **“what sorts of people were more likely to survive?”** using passenger data (ie name, age, gender, socio-economic class, etc). **You will have to submit your prediction on Kaggle**. |
||||
|
||||
## Preliminary |
||||
|
||||
The way the Kaggle platform works is explained in the challenge overview page. If you need more details, I suggest this [resource](https://towardsdatascience.com/getting-started-with-kaggle-f9138b35ae18) that gives detailed explanations. |
||||
|
||||
- Create a username following this structure: username_01EDU_ location_MM_YYYY. Submit the description profile and push it on GitHub the first day of the week. Do not touch this file anymore. |
||||
|
||||
- It is possible to have different personal accounts merged in a team for one single competition. |
||||
|
||||
## Deliverables |
||||
|
||||
```console |
||||
project |
||||
│ README.md |
||||
│ environment.yml |
||||
│ username.txt |
||||
│ |
||||
└───data |
||||
│ │ train.csv |
||||
│ | test.csv |
||||
| | gender_submission.csv |
||||
│ |
||||
└───notebook |
||||
│ │ EDA.ipynb |
||||
| |
||||
|───scripts |
||||
│ |
||||
|
||||
``` |
||||
|
||||
- `README.md` introduction of the project, shows the username, describes the features engineering and the best score on the **leaderboard**. Note the score on the test set using the exact same pipeline that led to the best score on the leaderboard. |
||||
|
||||
- `environment.yml` contains all libraries required to run the code. |
||||
|
||||
- `username.txt` contains the username, the last modified date of the file **has to correspond to the first day of the project**. |
||||
|
||||
- `EDA.ipynb` contains the exploratory data analysis. This file should contain all steps of data analysis that contributed or not to improve the accuracy. It has to be commented so that the reviewer can understand the analysis and run it without any problem. |
||||
|
||||
- `scripts` contains python file(s) that perform(s) the feature engineering, the model's training and prediction on the test set. It could also be one single Jupyter Notebook. It has to be commented to help the reviewers understand the approach and run the code without any bugs. |
||||
- **Submit your predictions on the Kaggle's competition platform**. Check your ranking and score in the leaderboard. |
||||
|
||||
### Scores |
||||
|
||||
In order to validate the project you will have to score at least **79% accuracy on the Leaderboard**: |
||||
|
||||
- 79% accuracy is the minimum score to validate the project. |
||||
|
||||
Scores indication: |
||||
|
||||
- 79% difficult - minimum required |
||||
- 81% very difficult: smart feature engineering needed |
||||
- More than 83%: excellent that corresponds to the top 2% on Kaggle |
||||
- More than 85%: cheating |
||||
|
||||
#### Cheating |
||||
|
||||
It is impossible to get 100%. Who would have predicted that Rose wouldn't let [Jack on the door](https://www.insider.com/jack-and-rose-werent-on-a-door-in-titanic-2019-7) ? |
||||
|
||||
All people having 100% of accuracy on the Leaderboard cheated, there's no point to compare with them or to cheat. The Kaggle community estimates that having more than 85% is almost considered as cheated submissions as they are element of luck involved in the surviving. |
||||
|
||||
**You can't used external data sets than the ones provided in that competition.** |
||||
|
||||
## The key points |
||||
|
||||
- **Feature engineering**: |
||||
Put yourself in the shoes of an investigator trying to understand what happened exactly in that boat during the crash. Do not hesitate to watch the movie to try to find as many insights as possible. Without a smart the feature engineering there's no way to validate the project ;-) |
||||
|
||||
- The leaderboard evaluates on test data for which you don't have the labels. It means that there's no point to over fit the train set. Check the over fitting on the train set by dividing the data and by cross-validating the accuracy. |
||||
|
||||
## Advice |
||||
|
||||
Don't try to build the perfect model the first day. Iterate a lot and test your assumptions: |
||||
|
||||
Iteration 1: |
||||
|
||||
- Predict all passengers die |
||||
|
||||
Iteration 2 |
||||
|
||||
- Fit a logistic regression with a basic feature engineering |
||||
|
||||
Iteration 3: |
||||
|
||||
- Perform an EDA. Make assumptions and check them. Example: What if first class passengers survived more. Check the assumption through EDA and create relevant features to help the model capture the information. |
||||
|
||||
- Run a gridsearch |
||||
|
||||
Iteration 4: |
||||
|
||||
- Good luck ! |
Before Width: | Height: | Size: 71 KiB |
@ -1,109 +0,0 @@
|
||||
# Project02 - NLP-enriched News Intelligence platform - audit |
||||
|
||||
### Preliminary |
||||
|
||||
``` |
||||
project |
||||
│ README.md |
||||
│ environment.yml |
||||
│ |
||||
└───data |
||||
│ │ topic_classification_data.csv |
||||
│ |
||||
└───results |
||||
│ │ topic_classifier.pkl |
||||
│ │ learning_curves.png |
||||
│ │ enhanced_news.csv |
||||
| |
||||
|───nlp_engine |
||||
│ |
||||
|
||||
``` |
||||
|
||||
###### Does the structure of the project is as below ? |
||||
|
||||
###### Does the readme file give an introduction of the project, show the username, describe the feature engineering and show the best score the on the leaderboard ? |
||||
|
||||
###### Does the environment contain all libraries used and their versions that are necessary to run the code ? |
||||
|
||||
|
||||
|
||||
|
||||
### Scrapper |
||||
|
||||
##### There are at least 300 news articles stored in the file system or the database. |
||||
##### Run the scrapper with `python scrapper_news.py` and fetch 3 documents. The scrapper is not expected to fetch 3 documents and stop by itself, you can stop it manually. It runs without any error and stores the 3 files as expected. |
||||
|
||||
### Topic classifier |
||||
|
||||
###### Are the learning curves provided ? |
||||
###### Do the learning curves prove the topics classifier is trained without correctly - without overfitting ? |
||||
###### Can you run the topic classfier model on the test set without any error ? |
||||
###### Does the topic classifier score an accuracy higher than 95% ? |
||||
|
||||
### Scandal detection |
||||
|
||||
###### Does the `README.md` explain the choice of embeddings and distance ? |
||||
|
||||
###### Does the DataFrame flag the top 10 articles with the highest likelihood of environmental scandal ? |
||||
|
||||
###### Is the distance or similarity saved in the DataFrame ? |
||||
|
||||
##### |
||||
### NLP engine output on 300 articles |
||||
|
||||
###### Does the DataFrame contain 300 different rows ? |
||||
###### Does the columns of the DataFrame are as expected ? |
||||
|
||||
``` |
||||
Date scrapped (date) |
||||
Title (str) |
||||
URL (str) |
||||
Body (str) |
||||
Org (str) |
||||
Topics (list str) |
||||
Sentiment (list float or float) |
||||
Scandal_distance (float) |
||||
Top_10 (bool) |
||||
|
||||
``` |
||||
|
||||
##### Analyse the DataFrame with 300 articles: relevance of the topics matched, relevance of the sentiment, relevance of the scandal detected and relevance of the companies matched. The algorithms are not 100% accurate so you should expect a few issues in the results. |
||||
|
||||
|
||||
### NLP engine on 3 articles |
||||
|
||||
###### Can you run `python nlp_enriched_news.py` without any error ? |
||||
|
||||
###### Does the output of the nlp engine correspond to the output below? |
||||
|
||||
```prompt |
||||
python nlp_enriched_news.py |
||||
|
||||
Enriching <URL>: |
||||
|
||||
Cleaning document ... (optional) |
||||
|
||||
---------- Detect entities ---------- |
||||
|
||||
Detected <X> companies which are <company_1> and <company_2> |
||||
|
||||
---------- Topic detection ---------- |
||||
|
||||
Text preprocessing ... |
||||
|
||||
The topic of the article is: <topic> |
||||
|
||||
---------- Sentiment analysis ---------- |
||||
|
||||
Text preprocessing ... (optional) |
||||
The title which is <title> is <sentiment> |
||||
The body of the article is <sentiment> |
||||
|
||||
---------- Scandal detection ---------- |
||||
|
||||
Computing embeddings and distance ... |
||||
|
||||
Environmental scandal detected for <entity> |
||||
``` |
||||
##### Analyse the output: relevance of the topic(s) matched, relevance of the sentiment, relevance of the scandal detected (if detected on the three articles) and relevance of the companie(s) matched. |
@ -1,180 +0,0 @@
|
||||
# NLP-enriched News Intelligence platform |
||||
|
||||
The goal of this project is to build an NLP-enriched News Intelligence platform. News analysis is a trending and important topic. The analysts get their information from the news and the amount of available information is limitless. Having a platform that helps to detect the relevant information is definitely valuable. |
||||
|
||||
|
||||
The platform connects to a news data source, detects the entities, detects the topic of the article, analyse the sentiment and ... |
||||
|
||||
## Scrapper |
||||
|
||||
News data source: |
||||
|
||||
- Find a news website that is easy to scrap. I could have chosen the website but the news' websites change their scraping policy frequently. |
||||
- Store it: |
||||
- File system per day: |
||||
- URL, date unique id |
||||
- headline |
||||
- body of the article |
||||
- SQL database (optional) |
||||
|
||||
from the last week otherwise the volume may be to high |
||||
|
||||
|
||||
## NLP engine |
||||
|
||||
In production architectures, the NLP engine delivers a live output based on the news that are delivered in a live stream data by the scrapper. However, it required advanced Python skills that are not a pre-requisite for the AI branch. |
||||
To simplify this step the scrapper and the NLP engine are used independently in the project. The scrapper fetches the news and store them in the data structure (either the file systeme or the SQL database) and then, the NLP engine runs on the stored data. |
||||
|
||||
Here how the NLP engine should process the news: |
||||
|
||||
### **1. Entities detection:** |
||||
|
||||
The goal is to detect all the entities in the document (headline and body). The type of entity we focus on is `ORG`. This corresponds to companies and organisations. This information should be stored. |
||||
|
||||
- Detect all companies using SpaCy NER on the body of the text. |
||||
|
||||
https://towardsdatascience.com/named-entity-recognition-with-nltk-and-spacy-8c4a7d88e7da |
||||
|
||||
|
||||
### **2. Topic detection:** |
||||
|
||||
The goal is to detect what the article is dealing with: Tech, Sport, Business, Entertainment or Politics. To do so, a labelled dataset is provided. From this dataset, build a classifier that learns to detect the right topic in the article. The trained model should be stored as `topic_classifier.pkl`. Make sure the model can be used easily (with the preprocessing pipeline built for instance) because the audit requires the auditor to test the model. |
||||
|
||||
Save the plot of learning curves (`learning_curves.png`) in `results` to prove that the model is trained correctly and not overfitted. |
||||
|
||||
- Learning constraints: **Score on test: > 95%** |
||||
|
||||
- **Optional**: If you want to train a news' topic classifier based on a more challenging dataset, you can use the following which based on 200k news headlines. https://www.kaggle.com/rmisra/news-category-dataset. |
||||
|
||||
|
||||
### **3. Sentiment analysis:** |
||||
|
||||
The goal is to detect the sentiment of the news articles. To do so, use a pre-trained sentiment model. I suggest to use: NLTK. |
||||
There are 3 reasons for which we use a pre-trained model: |
||||
|
||||
1. As a Data Scientist, you should learn to use a pre-trained model. There are so many models available and trained that sometimes you don't need to train one from scratch. |
||||
2. Labelled news data for sentiment analysis are very expensive. Companies as SESAMm provide this kind of services. |
||||
3. You already know how to train a sentiment analysis classifier ;-) |
||||
|
||||
### **4. Scandal detection ** |
||||
|
||||
The goal is to detect environmental disaster for the detected companies. Here is the methodology that should be used: |
||||
- Define keywords that correspond to environmental disaster that may be caused by companies: pollution, deforestation etc ... Here is an example of disaster we want to detect: https://en.wikipedia.org/wiki/MV_Erika. Pay attention to not use ambigous words that make sense in the context of an environmental disaster but also in another context. This would lead to detect a false positive natural disaster. |
||||
|
||||
- Compute the embeddings of the keywords. |
||||
|
||||
- Compute the distance between the embeddings of the keywords and all sentences that contain an entity. Explain in the `README.md` the embeddings chosen and why. Similarly explain the distance or similarity chosen and why. |
||||
|
||||
- Save the distance |
||||
|
||||
- Flag the top 10 articles. |
||||
|
||||
### 5. **Source analysis (optional)** |
||||
|
||||
The goal is to show insights about the news' source you scrapped. |
||||
This requires to scrap data on at least 5 days (a week ideally). Save the plots in the `results` folder. |
||||
|
||||
Here are examples of insights: |
||||
|
||||
- Per day: |
||||
|
||||
- Proportion of topics per day |
||||
- Number of articles |
||||
- Number of companies mentioned |
||||
- Sentiment per day |
||||
|
||||
- Per companies: |
||||
|
||||
- Companies mentioned the most |
||||
- Sentiment per companies |
||||
|
||||
|
||||
## Deliverables |
||||
|
||||
The structure of the project is: |
||||
|
||||
``` |
||||
project |
||||
│ README.md |
||||
│ environment.yml |
||||
│ |
||||
└───data |
||||
│ │ topic_classification_data.csv |
||||
│ |
||||
└───results |
||||
│ │ topic_classifier.pkl |
||||
│ │ learning_curves.png |
||||
│ │ enhanced_news.csv |
||||
| |
||||
|───nlp_engine |
||||
│ |
||||
|
||||
``` |
||||
|
||||
1. Run the scrapper until it fetches at least 300 articles |
||||
|
||||
``` |
||||
python scrapper_news.py |
||||
|
||||
1. scrapping <URL> |
||||
requesting ... |
||||
parsing ... |
||||
saved in <path> |
||||
|
||||
2. scrapping <URL> |
||||
requesting ... |
||||
parsing ... |
||||
saved in <path> |
||||
|
||||
``` |
||||
|
||||
2. Run on this 300 articles the NLP engine. |
||||
|
||||
Save a DataFrame: |
||||
|
||||
Date scrapped (date) |
||||
Title (str) |
||||
URL (str) |
||||
Body (str) |
||||
Org (str) |
||||
Topics (list str) |
||||
Sentiment (list float or float) |
||||
Scandal_distance (float) |
||||
Top_10 (bool) |
||||
|
||||
```prompt |
||||
python nlp_enriched_news.py |
||||
|
||||
Enriching <URL>: |
||||
|
||||
Cleaning document ... (optional) |
||||
|
||||
---------- Detect entities ---------- |
||||
|
||||
Detected <X> companies which are <company_1> and <company_2> |
||||
|
||||
---------- Topic detection ---------- |
||||
|
||||
Text preprocessing ... |
||||
|
||||
The topic of the article is: <topic> |
||||
|
||||
---------- Sentiment analysis ---------- |
||||
|
||||
Text preprocessing ... (optional) |
||||
The title which is <title> is <sentiment> |
||||
The body of the article is <sentiment> |
||||
|
||||
---------- Scandal detection ---------- |
||||
|
||||
Computing embeddings and distance ... |
||||
|
||||
Environmental scandal detected for <entity> |
||||
``` |
||||
|
||||
I strongly suggest to create a data structure (dictionary for example) to save all the intermediate result. Then, a boolean argument `cache` fetched the intermediate results when they are already computed. |
||||
|
||||
|
||||
Ressources: |
||||
|
||||
- https://www.youtube.com/watch?v=XVv6mJpFOb0 |
@ -1,131 +0,0 @@
|
||||
# Project03 - Computer vision - audit |
||||
|
||||
### Preliminary |
||||
|
||||
|
||||
``` |
||||
project |
||||
│ README.md |
||||
│ environment.yml |
||||
│ |
||||
└───data |
||||
│ │ train.csv |
||||
│ │ test.csv |
||||
│ │ xxx.csv |
||||
│ |
||||
└───results |
||||
│ │ |
||||
| |───model (free format) |
||||
│ │ │ my_own_model.pkl |
||||
│ │ │ my_own_model_architecture.txt |
||||
│ │ │ tensorboard.png |
||||
│ │ │ learning_curves.png |
||||
│ │ │ pre_trained_model.pkl (optional) |
||||
│ │ │ pre_trained_model_architecture.txt (optional) |
||||
│ │ |
||||
| |───hack_cnn (free format) |
||||
│ │ │ hacked_image.png (optional) |
||||
│ │ │ input_image.png |
||||
│ │ |
||||
| |───preprocessing_test |
||||
| | | input_video.mp4 (free format) |
||||
│ │ │ image0.png (free format) |
||||
│ │ │ image1.png |
||||
│ │ │ imagen.png |
||||
│ │ │ image20.png |
||||
| |
||||
|───scripts |
||||
│ │ train.py |
||||
│ │ predict.py |
||||
│ │ preprocess.py |
||||
│ │ predict_live_stream.py |
||||
│ │ hack_the_cnn.py |
||||
|
||||
``` |
||||
|
||||
###### Does the structure of the project is as below ? |
||||
|
||||
###### Does the readme file summurize how to run the code and explain the global approach ? |
||||
|
||||
###### Does the environment contain all libraries used and their versions that are necessary to run the code ? |
||||
|
||||
###### Do the text files explain the chosen architectures ? |
||||
|
||||
|
||||
|
||||
### CNN emotion classifier |
||||
|
||||
|
||||
###### Is the model trained only the training set ? |
||||
###### Is the accuracy on the test set is higher than 70% ? |
||||
###### Do the learning curves prove the model the model is not overfitting ? |
||||
###### Has the training been stopped early enough to avoid the overfitting ? |
||||
###### Does the screenshot show the usage of the tensorboard to monitor the training ? |
||||
###### Does the text document explain why the architecture was chosen and what were the previous iterations ? |
||||
###### Does the following command `python predict.py ` run without any error and returns an accuracy greater than 70% ? |
||||
|
||||
```prompt |
||||
python predict.py |
||||
|
||||
Accuracy on test set: 72% |
||||
|
||||
``` |
||||
|
||||
|
||||
### Face detection on the video stream |
||||
|
||||
|
||||
###### Does the preprocessing pipeline take as input the webcam video stream of minimum 20 sec and save in a separate folder at least 20 preprocessed* images ? |
||||
|
||||
###### Do all images contain a face ? |
||||
|
||||
###### Are all images reshaped and centered on the face ? |
||||
|
||||
###### Is the algorithm that detects the face imported via cv2 ? |
||||
|
||||
###### Is the image converted to 48 x 48 grayscale pixels' image |
||||
|
||||
###### If there's an issue related to the webcam, does the code takes as input a video recorded video stream ? |
||||
|
||||
###### Does the following command `predict_live_stream.py` run without any error and return the following ? |
||||
|
||||
```prompt |
||||
python predict_live_stream.py |
||||
|
||||
Reading video stream ... |
||||
|
||||
Preprocessing ... |
||||
11:11:11s : Happy , 73% |
||||
|
||||
Preprocessing ... |
||||
11:11:12s : Happy , 93% |
||||
|
||||
Preprocessing ... |
||||
11:11:13s : Surprise , 71% |
||||
|
||||
Preprocessing ... |
||||
11:11:14s : Neutral , 82% |
||||
|
||||
... |
||||
|
||||
Preprocessing ... |
||||
11:13:29s : Happy , 63% |
||||
|
||||
``` |
||||
|
||||
|
||||
### Hack the CNN - guidelines: |
||||
|
||||
The neural network trains by updating its weights given the training error. If an image is misclassfied the neural network changes its weight to classify it correctly. The trick is to keep the neural network's weights unchanged and to modify the input pixels in order to force the neural network to predict the wanted class. |
||||
This part is validated if: |
||||
|
||||
##### Choose an image from the database that gives more than 90% probability of `Happy` |
||||
###### Does the neural network modifies the input pixels to predict Sad ? |
||||
###### Can you recognize easily the chosen image ? The modified image is SLIGHTLY changed. It means that you recognies very easily the original image. |
||||
|
||||
|
||||
|
||||
Here are three ressources that detail similar approaches: |
||||
- https://github.com/XC-Li/Facial_Expression_Recognition/tree/master/Code/RAFDB |
||||
- https://github.com/karansjc1/emotion-detection/tree/master/with%20flask |
||||
- https://www.kaggle.com/drbeanesp21/aliaj-final-facial-expression-recognition (simplified) |
@ -1,156 +0,0 @@
|
||||
# Emotions detection with Deep Learning |
||||
Cameras are everywhere. Videos and images have become one of the most interesting data sets for artificial intelligence. |
||||
Image processing is a quite board research area, not just filtering, compression, and enhancement. Besides, we are even interested in the question, “what is in images?”, i.e., content analysis of visual inputs, which is part of the main task of computer vision. The study of computer vision could make possible such tasks as 3D reconstruction of scenes, motion capturing, and object recognition, which are crucial for even higher-level intelligence such as |
||||
image and video understanding, and motion understanding. |
||||
For this 2 months project we will focus on two tasks: |
||||
|
||||
- emotion classfication |
||||
- face tracking |
||||
|
||||
With the computing power exponentially increasing the computer vision field has been developping exponentially. This is a key element because the computer power allows to use more easily a type of neural networks very powerful on images: CNN's (Convolutional Neural Networks). Before the CNN's were democratized, the algorithms used relied a lot on human analysis to extract features which obviously time consuming and not reliable. If you're interested in the "old school methodology" this article explains it: towardsdatascience.com/classifying-facial-emotions-via-machine-learning-5aac111932d3. |
||||
The history behind this field is fascinating ! Here is a short summary of its history https://kapernikov.com/basic-introduction-to-computer-vision/ |
||||
|
||||
## Project goal and suggested timeline |
||||
|
||||
The goal of the project is to implement a **system that detects the emotion on a face from a webcam video stream**. To achieve this exciting task you'll have to understand how to: |
||||
|
||||
- deal with images in Python |
||||
- detect a face in an image |
||||
- train a CNN to detect the emotion on a face |
||||
|
||||
That is why I suggest to start the project with a preliminary step. The goal of this step is to understand how CNNs work and how to classify images. This preliminary step should take approximately **two weeks**. |
||||
|
||||
Then starts the emotion detection in a webcam video stream step that will last until the end of the project ! |
||||
|
||||
The two steps are detailed below. |
||||
|
||||
|
||||
## Preliminary: |
||||
|
||||
- Take this lesson. This course is a reference for many reasons and one of them is the creator: **Andrew Ng**. He explains the basics of CNNs but also some more advanced topics as transfer learning, siamese networks etc ... I suggest to focus on Week 1 and 2 and to spend less time on Week 3 and 4. Don't worry the time scoping of such MOOCs are conservative ;-). Here is the link: https://www.coursera.org/learn/convolutional-neural-networks . You can attend the lessons for free ! |
||||
|
||||
- Participate to this challenge: https://www.kaggle.com/c/digit-recognizer/code . The MNIST dataset is a reference in computer vision. Researchers use it as a benchmark to compare their models. Start first with a logistic regression to understand how to handle images in Python. And then train your first CNN on this data set. |
||||
|
||||
|
||||
## Face emotions classification |
||||
|
||||
|
||||
Emotion detection is one of the most researched topics in the modern-day machine learning arena. The ability to accurately detect and identify an emotion opens up numerous doors for Advanced Human Computer Interaction. The aim of this project is to detect up to seven distinct facial emotions in real time. This project runs on top of a Convolutional Neural Network (CNN) that is built with the help of Keras whose backend is TensorFlow in Python. The facial emotions that can be detected and classified by this system are Happy, Sad, Angry, Surprise, Fear, Disgust and Neutral. |
||||
|
||||
|
||||
Your goal is to implement a program that takes as input a video stream that contains a person's face and that predicts the emotion of the person. |
||||
|
||||
**Step 1**: **Fit the emotion classifier** |
||||
|
||||
- Train a CNN on the dataset `train.csv`. Here is an example of architecture you can implement: https://www.quora.com/What-is-the-VGG-neural-network . **The CNN has to perform more than 70% on the test set**. You will see that the CNNs take a lot of time to train. You don't want to overfit the neural network. I strongly suggest to use early stopping, callbacks and to monitor the training using the tensorboard. |
||||
|
||||
You have to save the trained model in `my_own_model.pkl` and to explain the chosen architecture in `my_own_model_architecture.txt`. Use `model.summary())` to print the architecture. It is also expected that you explains the iterations and how you end up choosing your final architecture. Save a screenshot of the tensorboard while the model's training in `tensorboard.png` and save a plot with the learning curves showing the model training and stopping BEFORE the model starts overfitting in `learning_curves.png`. |
||||
|
||||
- Optional: Use a pre-trained CNN to improve the accuracy. You will find some huge CNN's architecture that perform well. The issue is that it is expensive to train them from scratch. You'll need a lot of GPUs, memory and time. **Pre-trained CNNs** solve partially this issue because they are already trained on a dataset and perform well on some use cases. However, building a CNN from scratch is required, as mentioned, this step is optional and doesn't replace the first one. Similarly, save the model and explain the chosen architecture. |
||||
|
||||
**Step 2**: **Classify emotions from a video stream** |
||||
|
||||
|
||||
- Use the video stream outputted by your computer's webcam and preprocess it to make it compatible with the CNN you trained. One of the preprocessing steps is: face detection. As you may have seen the training samples are imaged centered on a face. To do so, I suggest to use a pre-trained model to detect faces. OpenCV for image processing tasks where we identify a face from a live webcam feed which is then processed and fed into the trained neural network for emotion detection. The preprocessing pipeline will be corrected with a functional test in `preprocessing_test`: |
||||
- **Input**: Video stream of 20 sec with a face on it |
||||
- **Output**: 20 (or 21) images cropped and centered on the face with 48 x 48 grayscale pixels |
||||
|
||||
- Predict at least one emotion per second from the video stream. The minimum requirement is printing in the prompt the predicted emotion with its associated probability. If there's any problem related to the webcam use as input the a recorded video stream. |
||||
|
||||
For that step, I suggest again to use **OpenCV** as much as possible: |
||||
|
||||
- https://opencv-python-tutroals.readthedocs.io/en/latest/py_tutorials/py_gui/py_video_display/py_video_display.html |
||||
|
||||
|
||||
- Optional: **(very cool)** Hack the CNN. Take a picture for which the prediction of your CNN is **Happy**. Now, hack the CNN: using the same image **SLIGHTLY** modified make the CNN predict **Sad**. https://medium.com/@ageitgey/machine-learning-is-fun-part-8-how-to-intentionally-trick-neural-networks-b55da32b7196 |
||||
|
||||
|
||||
## Deliverable |
||||
|
||||
|
||||
``` |
||||
project |
||||
│ README.md |
||||
│ environment.yml |
||||
│ |
||||
└───data |
||||
│ │ train.csv |
||||
│ │ test.csv |
||||
│ │ xxx.csv |
||||
│ |
||||
└───results |
||||
│ │ |
||||
| |───model (free format) |
||||
│ │ │ my_own_model.pkl |
||||
│ │ │ my_own_model_architecture.txt |
||||
│ │ │ tensorboard.png |
||||
│ │ │ learning_curves.png |
||||
│ │ │ pre_trained_model.pkl (optional) |
||||
│ │ │ pre_trained_model_architecture.txt (optional) |
||||
│ │ |
||||
| |───hack_cnn (free format) |
||||
│ │ │ hacked_image.png (optional) |
||||
│ │ │ input_image.png |
||||
│ │ |
||||
| |───preprocessing_test |
||||
| | | input_video.mp4 (free format) |
||||
│ │ │ image0.png (free format) |
||||
│ │ │ image1.png |
||||
│ │ │ imagen.png |
||||
│ │ │ image20.png |
||||
| |
||||
|───scripts |
||||
│ │ train.py |
||||
│ │ predict.py |
||||
│ │ preprocess.py |
||||
│ │ predict_live_stream.py |
||||
│ │ hack_the_cnn.py |
||||
|
||||
``` |
||||
|
||||
- Run **predict.py** expected output: |
||||
|
||||
```prompt |
||||
python predict.py |
||||
|
||||
Accuracy on test set: 72% |
||||
|
||||
``` |
||||
- Run **predict_live_stream.py** expected output: |
||||
|
||||
```prompt |
||||
python predict_live_stream.py |
||||
|
||||
Reading video stream ... |
||||
|
||||
Preprocessing ... |
||||
11:11:11s : Happy , 73% |
||||
|
||||
Preprocessing ... |
||||
11:11:12s : Happy , 93% |
||||
|
||||
Preprocessing ... |
||||
11:11:13s : Surprise , 71% |
||||
|
||||
Preprocessing ... |
||||
11:11:14s : Neutral , 82% |
||||
|
||||
... |
||||
|
||||
Preprocessing ... |
||||
11:13:29s : Happy , 63% |
||||
|
||||
``` |
||||
|
||||
## Useful ressources: |
||||
|
||||
- https://machinelearningmastery.com/what-is-computer-vision/ |
||||
|
||||
- Use a pre-trained CNN: https://arxiv.org/pdf/1812.06387.pdf |
||||
|
||||
- Hack the CNN https://medium.com/@ageitgey/machine-learning-is-fun-part-8-how-to-intentionally-trick-neural-networks-b55da32b7196 |
||||
|
||||
- http://ice.dlut.edu.cn/valse2018/ppt/WeihongDeng_VALSE2018.pdf |
||||
|
||||
- https://arxiv.org/pdf/1812.06387.pdf |
||||
|
Before Width: | Height: | Size: 61 KiB |
@ -1,133 +0,0 @@
|
||||
# Financial strategies on the SP500 |
||||
|
||||
This documents is the correction of the project 4. Some steps are detailed in W1D5E4. TODO: replace with quest name |
||||
|
||||
|
||||
``` |
||||
project |
||||
│ README.md |
||||
│ environment.yml |
||||
│ |
||||
└───data |
||||
│ │ sp500.csv |
||||
│ |
||||
└───results |
||||
│ │ |
||||
| |───cross-validation |
||||
│ │ │ ml_metrics_train.csv |
||||
│ │ │ metric_train.csv |
||||
│ │ │ top_10_feature_importance.csv |
||||
│ │ │ metric_train.png |
||||
│ │ |
||||
| |───selected model |
||||
│ │ │ selected_model.pkl |
||||
│ │ │ selected_model.txt |
||||
│ │ │ ml_signal.csv |
||||
│ │ |
||||
| |───strategy |
||||
| | | strategy.png |
||||
│ │ │ results.csv |
||||
│ │ │ report.md |
||||
| |
||||
|───scripts (free format) |
||||
│ │ features_engineering.py |
||||
│ │ gridsearch.py |
||||
│ │ model_selection.py |
||||
│ │ create_signal.py |
||||
│ │ strategy.py |
||||
|
||||
``` |
||||
|
||||
###### Does the structure of the project is as below ? |
||||
|
||||
###### Does the readme file summurize how to run the code and explain the global approach ? |
||||
|
||||
###### Does the environment contain all libraries used and their versions that are necessary to run the code ? |
||||
|
||||
###### Do the text files explain the chosen model methodology ? |
||||
|
||||
|
||||
## **Data processing and feature engineering** |
||||
|
||||
###### Is the data splitted in a train set and test set ? |
||||
###### Is the last day of the train set is D and the first day of the test set is D+n with n>0 ? Splitting without considering the time series structure is wrong. |
||||
|
||||
##### There is no leakage: unfortunately there's no autamated way to check if the dataset is leaked. This step is validated if the features of date d are built as follow: |
||||
|
||||
| Index | Features |Target | |
||||
|----------|:-------------: |------:| |
||||
| Day D-1 | Features until D-1 23:59pm | return(D, D+1) | |
||||
| Day D | Features until D 23:59pm | return(D+1, D+2) | |
||||
| Day D+1 | Features until D+1 23:59pm | return(D+2, D+3) | |
||||
|
||||
###### Have the features been grouped by ticker before to compute the features ? |
||||
|
||||
###### - Has the target been grouped by ticker before to compute the futur returns ? |
||||
|
||||
|
||||
## **Machine Learning pipeline** |
||||
|
||||
### Cross-Validation |
||||
|
||||
###### Does the CV contain at least 10 folds in total ? |
||||
###### Do all train folds have more than 2y history ? If you use time series split, checking that the first fold has more than 2y history is enough. |
||||
##### The last validation set of the train set doesn't overlap on the test set. |
||||
##### None of the folds contain data from the same day.The split should be done on the dates. |
||||
##### There's a plot showing your cross-validation. As usual, all plots should have named axis and a title.If you chose a Time Series Split the plot should look like this: |
||||
|
||||
|
||||
![alt text][timeseries] |
||||
|
||||
[timeseries]: ../Time_series_split.png "Time Series split" |
||||
|
||||
|
||||
### Model Selection |
||||
|
||||
##### The test set hasn't been used to train the model and select the model. |
||||
###### Is the selected model saved in the pkl file and described in a txt file ? |
||||
|
||||
### Selected model |
||||
|
||||
##### The ml metrics computed on the train set are agregated: sum or median. |
||||
###### Are the ml metrics saved in a csv file ? |
||||
###### Are the top 10 important features per fold are saved in `top_10_feature_importance.csv`? |
||||
###### Does `metric_train.png` show a plot similar to the one below ? |
||||
*Note that, this can be done also on the test set **IF** this hasn't helped to select the pipeline. * |
||||
|
||||
![alt text][barplot] |
||||
|
||||
[barplot]: ../metric_plot.png "Metric plot" |
||||
|
||||
### Machine learning signal |
||||
|
||||
##### **The pipeline shouldn't be trained once and predict on all data points !** As explained: The signal has to be generated with the chosen cross validation: train the model on the train set of the first fold, then predict on its validation set; train the model on the train set of the second fold, then predict on its validation set, etc ... Then, concatenate the predictions on the validation sets to build the machine learning signal. |
||||
|
||||
|
||||
## **Strategy backtesting** |
||||
|
||||
### Convert machine learning signal into a strategy |
||||
|
||||
##### The transformed machine learning signal (long only, long short, binary, ternary, stock picking, proportional to probability or custom ) is multiplied by the return between d+1 and d+2. As a reminder, the signal at date d predicts wether the return between d+1 and d+2 is increasing or deacreasing. Then, the PnL of date d could be associated with date d, d+1 or d+2. This is arbitrary and should impact the value of the PnL. |
||||
|
||||
##### You invest the same amount of money every day. One exception: if you invest 1$ per day per stock the amount invested every day may change depending on the strategy chosen. If you take into account the different values of capital invested every day in the calculation of the PnL, the step is still validated. |
||||
|
||||
### Metrics and plot |
||||
|
||||
###### Is the Pnl computed as: strategy * futur_return ? |
||||
###### Does the strategy give the amount invested at time t on asset i ? |
||||
|
||||
###### Does the plot `strategy.png` contains an x axis: date ? |
||||
###### Does the plot `strategy.png` contains a y axis1: PnL of the strategy at time t ? |
||||
###### Does the plot `strategy.png` contains a y axis2: PnL of the SP500 at time t ? |
||||
###### Does the plot `strategy.png` use the same scale for y axis1 and y axis2 ? |
||||
###### Does the plot `strategy.png` contains a vertical line that shows the separation between train set and test set ? |
||||
|
||||
### Report |
||||
|
||||
###### Does the report detail the features used ? |
||||
|
||||
###### Does the report detail the pipeline used (imputer, scaler, dimension reduction and model) ? |
||||
|
||||
###### Does the report detail the cross-validation used (length of train sets and validation sets and if possible the cross-validation plot) ? |
||||
|
||||
###### Does the report detail the strategy chosen (description, PnL plot and the strategy metrics on the train set and test set) ? |
Before Width: | Height: | Size: 68 KiB |
Before Width: | Height: | Size: 56 KiB |
@ -1,214 +0,0 @@
|
||||
# Financial strategies on the SP500 |
||||
|
||||
TODO: data delivery and choose train/test split date. |
||||
|
||||
In this project we will apply machine to finance. You are a Quant/Data Scientist and your goal is to create a financial strategy based on a signal outputted by a machine learning model that overperforms the [SP500](https://en.wikipedia.org/wiki/S%26P_500). |
||||
|
||||
|
||||
The Standard & Poors 500 Index is a collection of stocks intended to reflect the overall return characteristics of the stock market as a whole. The stocks that make up the S&P 500 are selected by market capitalization, liquidity, and industry. Companies to be included in the S&P are selected by the S&P 500 Index Committee, which consists of a group of analysts employed by Standard & Poor's. |
||||
The S&P 500 Index originally began in 1926 as the "composite index" comprised of only 90 stocks. According to historical records, the average annual return since its inception in 1926 through 2018 is approximately 10%–11%.The average annual return since adopting 500 stocks into the index in 1957 through 2018 is roughly 8%. |
||||
As a Quant Researcher, you may beat the SP500 one year or few years. The real challenge though is to beat the SP500 consistently over decades. That's what most hedge funds in the world are trying to do. |
||||
|
||||
|
||||
The project is divided in parts: |
||||
|
||||
- **Data processing and feature engineering**: Build a dataset: insightful features and the target |
||||
- **Machine Learning pipeline**: Train machine learning models on the dataset, select the best model and generate the machine learning signal. |
||||
- **Strategy backtesting**: Generate a strategy from the Machine Learning model output and backtest the strategy. As a reminder, the idea here is to see what would have performed the strategy if you would have invested. |
||||
|
||||
## Deliverables |
||||
|
||||
Do not forget to check the ressources of W1D5 and espcially W1D5E4. |
||||
TODO: replace by quest name and exercice number |
||||
|
||||
### Data processing and features engineering |
||||
- Split the data in train and test (TODO: choose the year - once the data is delivered) |
||||
- Your first priority is to build a dataset without leakage !!! NO LEAKAGE !!! |
||||
|
||||
**"No leakage" small guide:** |
||||
We assume it is day D and we want to take a position on the next h days on the next day. The position starts on day D+1 (included). To decide wether we take a short or long position the return between day D+1 and D+2 is computed and used as a target. Finally, as features on day contain information until day D 11:59pm, target need to be shifted. As a result, the final dataframe schema is: |
||||
|
||||
|
||||
| Index | Features |Target | |
||||
|----------|:-------------: |------:| |
||||
| Day D-1 | Features until D-1 23:59pm | return(D, D+1) | |
||||
| Day D | Features until D 23:59pm | return(D+1, D+2) | |
||||
| Day D+1 | Features until D+1 23:59pm | return(D+2, D+3) | |
||||
|
||||
**Note: This table is simplified, the index of your DataFrame is a multi-index with date and ticker.** |
||||
|
||||
- Features: |
||||
- Bollinger |
||||
- RSI |
||||
- MACD |
||||
**Note: you can use any library to compute these features, you don't need to implement all financial features from scratch.** |
||||
|
||||
- Target: |
||||
- On day D, the target is: **sign(return(D+1, D+2))** |
||||
|
||||
> Remark: The target used is the return computed on the price and not the price directly. There are statistical reasons for this choice - the price is not stationary. The consequence is that a machine learning model tends to overfit while training on not stationary data. |
||||
|
||||
### Machine learning pipeline |
||||
|
||||
- Cross-validation deliverables: |
||||
- Implements a cross validation with at least 10 folds. The train set has to be bigger than 2 years history. |
||||
- Two types of temporal cross-validations are required: |
||||
- Blocking (plot below) |
||||
- Time Series split (plot below) |
||||
- Make sure the last fold of the train set does not overlap on the test set. |
||||
- Make sure the folds do not contain data from the same day. The data should be split on the dates. |
||||
- Plot your cross validation as follow: |
||||
|
||||
![alt text][blocking] |
||||
|
||||
[blocking]: blocking_time_series_split.png 'Blocking Time Series split' |
||||
|
||||
![alt text][timeseries] |
||||
|
||||
[timeseries]: Time_series_split.png 'Time Series split' |
||||
|
||||
Once you'll have run the gridsearch on the cross validation (choose either Blocking or Time Series split), you'll select the best pipeline on the train set and save it as `selected_model.pkl` and `selected_model.txt` (pipeline hyper-parameters). |
||||
|
||||
**Note: You may observe that the selected model is not good after analyzing the ml metrics (ON THE TRAIN SET) and select another one. ** |
||||
|
||||
- ML metrics and feature importances on the selected pipeline on the train set only. |
||||
- DataFrame with a Machine learning metrics on train et validation sets on all folds of the train set. Suggested format: columns: ML metrics (AUC, Accuracy, LogLoss), rows: folds, train set and validation set (double index). Save it as `ml_metrics_train.csv` |
||||
- Plot. Choose the metric you want. Suggested: AUC Save it as `metric_train.png`. The plot below shows how the plot should look like. |
||||
- DataFrame with top 10 important features for each fold. Save it as `top_10_feature_importance.csv` |
||||
|
||||
![alt text][barplot] |
||||
|
||||
[barplot]: metric_plot.png 'Metric plot' |
||||
|
||||
- The signal has to be generated with the chosen cross validation: train the model on the train set of the first fold, then predict on its validation set; train the model on the train set of the second fold, then predict on its validation set, etc ... Then, concatenate the predictions on the validation sets to build the machine learning signal. **The pipeline shouldn't be trained once and predict on all data points !** |
||||
|
||||
**The output is a DataFrame or Series with a double index ordered with the probability the stock price for asset i increases between d+1 and d+2.** |
||||
|
||||
- (optional): [Train a RNN/LSTM](https://towardsdatascience.com/predicting-stock-price-with-lstm-13af86a74944). This a nice way to discover and learn about recurrent neural networks. But keep in mind that there are some new neural network architectures that seem to outperform recurrent neural networks: https://towardsdatascience.com/the-fall-of-rnn-lstm-2d1594c74ce0. |
||||
|
||||
|
||||
## Strategy backtesting |
||||
|
||||
- Backtesting module deliverables. The module takes as input a machine learning signal, convert it into a financial strategy. A financial strategy DataFrame gives the amount invested at time t on asset i. The module returns the following metrics on the train set and the test set. |
||||
- PnL plot: save it as `strategy.png` |
||||
- x axis: date |
||||
- y axis1: PnL of the strategy at time t |
||||
- y axis2: PnL of the SP500 at time t |
||||
- Use the same scale for y axis1 and y axis2 |
||||
- add a line that shows the separation between train set and test set |
||||
- Pnl |
||||
- Max drawdown. https://www.investopedia.com/terms/d/drawdown.asp |
||||
- (Optional): add other metrics as sharpe ratio, volatility, etc ... |
||||
- Create a markdown report that explains and save it as `report.md`: |
||||
- the features used |
||||
- the pipeline used |
||||
- imputer |
||||
- scaler |
||||
- dimension reduction |
||||
- model |
||||
- the cross-validation used |
||||
- length of train sets and validation sets |
||||
- cross-validation plot (optional) |
||||
- strategy chosen |
||||
- description |
||||
- PnL plot |
||||
- strategy metrics on the train set and test set |
||||
|
||||
### Example of strategies: |
||||
- Long only: |
||||
- Binary signal: |
||||
0: do nothing for one day on asset i |
||||
1: take a long position on asset i for 1 day |
||||
- Weights proportional to the machine learning signals |
||||
- invest x on asset i for on day |
||||
- Long and short: For those who search long short strategy on Google, don't get wrong, this has nothing to do with pair trading. |
||||
- Binary signal: |
||||
- -1: take a short position on asset i for 1 day |
||||
- 1: take a long position on asset i for 1 day |
||||
- Ternary signal: |
||||
- -1: take a short position on asset i for 1 day |
||||
- 0: do nothing for one day on asset i |
||||
- 1: take a long position on asset i for 1 day |
||||
|
||||
Notes: |
||||
|
||||
- Warning! When you don't invest on all stock as in the binary signal or the ternary signal, make sure that you are still investing 1$ per day! |
||||
|
||||
- In order to simplify the **short position** we consider that this is the opposite of a long position. Example: I take a short one AAPL stock and the price decreases by 20$ on one day. I earn 20$. |
||||
|
||||
- Stock picking: Take a long position on the k best assets (from the machine learning signal) and short the k worst assets regarding the machine learning signal. |
||||
|
||||
Here's an example on how to convert a machine learning signal into a financial strategy: |
||||
|
||||
- Input: |
||||
|
||||
| Date | Ticker|Machine Learning signal | |
||||
|--------|:----: |-----------:| |
||||
| Day D-1| AAPL | 0.55 | |
||||
| Day D-1| C | 0.36 | |
||||
| Day D | AAPL | 0.59 | |
||||
| Day D | C | 0.33 | |
||||
| Day D+1| AAPL | 0.61 | |
||||
| Day D+1| C | 0.33 | |
||||
|
||||
- Convert it into a binary long only strategy: |
||||
- Machine learning signal > 0.5 |
||||
|
||||
| Date | Ticker|Binary signal | |
||||
|--------|:----: |-----------:| |
||||
| Day D-1| AAPL | 1 | |
||||
| Day D-1| C | 0 | |
||||
| Day D | AAPL | 1 | |
||||
| Day D | C | 0 | |
||||
| Day D+1| AAPL | 1 | |
||||
| Day D+1| C | 0 | |
||||
|
||||
|
||||
!!! BE CAREFUL !!!THIS IS EXTREMELY IMPORTANT. |
||||
|
||||
- Multiply it with the associated return. |
||||
|
||||
Don't forget the meaning of the signal on day d: it gives the return between d+1 and d+2. You should multiply the binary signal of day by the return computed between d+1 and d+2. Otherwise it's wrong because you use your signal that gives you information on d+1 and d+2 on the past or present. The strategy is leaked ! |
||||
|
||||
**Assumption**: you have 1$ per day to invest in your strategy. |
||||
|
||||
|
||||
## Project repository structure: |
||||
|
||||
``` |
||||
project |
||||
│ README.md |
||||
│ environment.yml |
||||
│ |
||||
└───data |
||||
│ │ sp500.csv |
||||
│ |
||||
└───results |
||||
│ │ |
||||
| |───cross-validation |
||||
│ │ │ ml_metrics_train.csv |
||||
│ │ │ metric_train.csv |
||||
│ │ │ top_10_feature_importance.csv |
||||
│ │ │ metric_train.png |
||||
│ │ |
||||
| |───selected model |
||||
│ │ │ selected_model.pkl |
||||
│ │ │ selected_model.txt |
||||
│ │ │ ml_signal.csv |
||||
│ │ |
||||
| |───strategy |
||||
| | | strategy.png |
||||
│ │ │ results.csv |
||||
│ │ │ report.md |
||||
| |
||||
|───scripts (free format) |
||||
│ │ features_engineering.py |
||||
│ │ gridsearch.py |
||||
│ │ model_selection.py |
||||
│ │ create_signal.py |
||||
│ │ strategy.py |
||||
|
||||
``` |
||||
Note: `features_engineering.py` can be used in `gridsearch.py` |
||||
|
||||
|
@ -1,86 +0,0 @@
|
||||
# Credit scoring |
||||
|
||||
## Preliminary |
||||
|
||||
``` |
||||
project |
||||
│ README.md |
||||
│ environment.yml |
||||
│ |
||||
└───data |
||||
│ │ ... |
||||
│ |
||||
└───results |
||||
│ │ |
||||
| |───model (free format) |
||||
│ │ │ my_own_model.pkl |
||||
│ │ │ model_report.txt |
||||
│ │ |
||||
| |feature_engineering |
||||
│ │ │ EDA.ipynb |
||||
│ │ |
||||
| |───clients_outputs |
||||
| | | client1_correct_train.pdf (free format) |
||||
│ │ │ client2_wrong_train.pdf (free format) |
||||
│ │ │ client_test.pdf (free format) |
||||
│ │ |
||||
| |───dashboard (optional) |
||||
| | | dashboard.py (free format) |
||||
│ │ │ ... |
||||
| |
||||
|───scripts (free format) |
||||
│ │ train.py |
||||
│ │ predict.py |
||||
│ │ preprocess.py |
||||
``` |
||||
###### Does the structure of the project is as below ? |
||||
|
||||
###### Does the readme file introduce the project, summurize how to run the code and show the username ? |
||||
|
||||
###### Does the environment contain all libraries used and their versions that are necessary to run the code ? |
||||
|
||||
###### Does the `EDA.ipynb` explain in details the exploratory data analysis ? |
||||
|
||||
|
||||
## Machine learning model |
||||
|
||||
###### Is the model trained only the training set ? |
||||
###### Is the AUC on the test set is higher than 75% ? |
||||
###### Does the model learning curves prove that the model is not overfitting ? |
||||
###### Has the training been stopped early enough to avoid the overfitting ? |
||||
###### Does the text document `model_report.txt` describe the methodology used to train the machine learning model ? |
||||
###### Does `predict.py` run without any error and returns the following ? |
||||
|
||||
|
||||
```prompt |
||||
python predict.py |
||||
|
||||
AUC on test set: 0.76 |
||||
|
||||
``` |
||||
|
||||
This [article](https://medium.com/thecyphy/home-credit-default-risk-part-2-84b58c1ab9d5) gives a complete example of a good modelling approach: |
||||
|
||||
|
||||
## Model's interpretability |
||||
|
||||
### Feature importance: |
||||
|
||||
###### Are the importance of all features used by the model computed and showed in a visualisation ? |
||||
|
||||
###### Is the mapping between between the importance of the features and the features' name is correct ? You should be careful here to associate the right variables to the their feature importance. Sometimes, the preprocessing pipeline can remove some features during the features selection step for instance. |
||||
|
||||
|
||||
### Descriptive variables: |
||||
##### These are important to understand for example the age of the client. If the data could be scaled or modified in the preprocessing pipeline but the data visualised here should be "raw". This part is validated if the visualisations are computed for the 3 clients. |
||||
- visualisations that show at least 10 variables describing the client and its loan(s) |
||||
- visualisations that show the comparison between this client and other clients. |
||||
|
||||
##### SHAP values on the model are displayed through a summary plot that shows the important features and their impact on the target. This is optional if you have already computed the features importance. |
||||
|
||||
###### Do the 3 clients are selected as expected ? 2 clients from the train set (1 on which the model is correct and 1 on which the model's wrong) and 1 client from the test set. |
||||
|
||||
##### SHAP values on predictions are computed for the 3 clients. The force plot shows what variables contributes the most to the score. **Check that the score outputted by the force plot corresponds to the one outputted by the model.** |
||||
|
||||
|
||||
|
Before Width: | Height: | Size: 358 KiB |
@ -1,46 +0,0 @@
|
||||
# Credit scoring data description |
||||
|
||||
This file describes the available data for the project. |
||||
|
||||
 |
||||
|
||||
## application_{train|test}.csv |
||||
|
||||
This is the main table, broken into two files for Train (with TARGET) and Test (without TARGET). |
||||
Static data for all applications. One row represents one loan in our data sample. |
||||
|
||||
## bureau.csv |
||||
|
||||
All client's previous credits provided by other financial institutions that were reported to Credit Bureau (for clients who have a loan in our sample). |
||||
For every loan in our sample, there are as many rows as number of credits the client had in Credit Bureau before the application date. |
||||
|
||||
## bureau_balance.csv |
||||
|
||||
Monthly balances of previous credits in Credit Bureau. |
||||
This table has one row for each month of history of every previous credit reported to Credit Bureau – i.e the table has (#loans in sample * # of relative previous credits * # of months where we have some history observable for the previous credits) rows. |
||||
|
||||
## POS_CASH_balance.csv |
||||
|
||||
Monthly balance snapshots of previous POS (point of sales) and cash loans that the applicant had with Home Credit. |
||||
This table has one row for each month of history of every previous credit in Home Credit (consumer credit and cash loans) related to loans in our sample – i.e. the table has (#loans in sample * # of relative previous credits * # of months in which we have some history observable for the previous credits) rows. |
||||
|
||||
## credit_card_balance.csv |
||||
|
||||
Monthly balance snapshots of previous credit cards that the applicant has with Home Credit. |
||||
This table has one row for each month of history of every previous credit in Home Credit (consumer credit and cash loans) related to loans in our sample – i.e. the table has (#loans in sample * # of relative previous credit cards * # of months where we have some history observable for the previous credit card) rows. |
||||
|
||||
## previous_application.csv |
||||
|
||||
All previous applications for Home Credit loans of clients who have loans in our sample. |
||||
There is one row for each previous application related to loans in our data sample. |
||||
|
||||
|
||||
## installments_payments.csv |
||||
|
||||
Repayment history for the previously disbursed credits in Home Credit related to the loans in our sample. |
||||
There is a) one row for every payment that was made plus b) one row each for missed payment. |
||||
One row is equivalent to one payment of one installment OR one installment corresponding to one payment of one previous Home Credit credit related to loans in our sample. |
||||
|
||||
## HomeCredit_columns_description.csv |
||||
|
||||
This file contains descriptions for the columns in the various data files. |
@ -1,23 +0,0 @@
|
||||
##### The exercice is validated is all questions of the exercice are validated |
||||
|
||||
##### Activate the virtual environment. If you used `conda` run `conda activate ex00` |
||||
|
||||
###### Does the shell specify the name `ex00` of the environment on the left ? |
||||
|
||||
##### Run `python --version` |
||||
|
||||
###### Does it print `Python 3.8.x`? x could be any number from 0 to 9. |
||||
|
||||
##### Does `import jupyter` and `import numpy` run without any error ? |
||||
|
||||
###### Have you used the followingthe command `jupyter notebook --port 8891` ? |
||||
|
||||
###### Is there a file named `Notebook_ex00.ipynb` in the working directory ? |
||||
|
||||
###### Is the following markdown code executed in a markdown cell in the first cell ? |
||||
|
||||
``` |
||||
# H1 TITLE |
||||
## H2 TITLE |
||||
``` |
||||
###### Does the second cell contain `print("Buy the dip ?")` and return `Buy the dip ?` in the output section ? |
@ -1,62 +0,0 @@
|
||||
# W1D01 Piscine AI - Data Science |
||||
|
||||
## NumPy |
||||
The goal of this day is to understand practical usage of **NumPy**. **NumPy** is a commonly used Python data analysis package. By using **NumPy**, you can speed up your workflow, and interface with other packages in the Python ecosystem, like scikit-learn, that use **NumPy** under the hood. **NumPy** was originally developed in the mid 2000s, and arose from an even older package called Numeric. This longevity means that almost every data analysis or machine learning package for Python leverages **NumPy** in some way. |
||||
|
||||
## Exercises of the day |
||||
|
||||
- Exercise 0 Environment and libraries |
||||
- Exercise 1 Your first NumPy array |
||||
- Exercise 2 Zeros |
||||
- Exercise 3 Slicing |
||||
- Exercise 4 Random |
||||
- Exercise 5 Split, concatenate, reshape arrays |
||||
- Exercise 6 Broadcasting and Slicing |
||||
- Exercise 7 NaN |
||||
- Exercise 8 Wine |
||||
- Exercise 9 Football tournament |
||||
|
||||
## Virtual Environment |
||||
- Python 3.x |
||||
- NumPy |
||||
- Jupyter or JupyterLab |
||||
|
||||
*Version of NumPy I used to do the exercises: 1.18.1*. |
||||
I suggest to use the most recent one. |
||||
|
||||
## Ressources |
||||
|
||||
- https://medium.com/fintechexplained/why-should-we-use-NumPy-c14a4fb03ee9 |
||||
- https://numpy.org/doc/ |
||||
- https://jakevdp.github.io/PythonDataScienceHandbook/ |
||||
|
||||
|
||||
# Exercise 0 Environment and libraries |
||||
|
||||
The goal of this exercise is to set up the Python work environment with the required libraries and to learn to launch a `jupyter notebook`. Jupyter notebooks are very convenient as they allow to write and test code within seconds. However, it really easy to implement instable and not reproducible code using notebooks. Keep the notebook and the underlying code clean. An article below detail when the Notebook should be used. Notebook can be used for most of the exercices of the piscine as the goal is to experiment A LOT. But no worries, you'll be asked to build a more robust structure for all the projects. |
||||
|
||||
**Note:** For each quest, your first exercice will be to set up the virtual environment with the required libraries. |
||||
|
||||
I recommend to use: |
||||
|
||||
- the **last stable versions** of Python. However, for educational purpose you will install a specific version of Python in this exercise. |
||||
- the virtual environment you're the most confortable with. `virtualenv` and `conda` are the most used in Data Science. |
||||
- one of the most recents versions of the libraries required |
||||
|
||||
1. Create a virtual environment named `ex00`, with Python `3.8`, with the following libraries: `numpy`, `jupyter`. |
||||
|
||||
2. Launch a `jupyter notebook` on port `8891` and create a notebook named `Notebook_ex00`. `JupyterLab` can be used instead of Jupyter Notebook here. |
||||
|
||||
3. Put the text `H1 TITLE` as **heading level 1** and `H2 TITLE` as **heading level 2** in the first cell. |
||||
|
||||
4. Run `print("Buy the dip ?")` in the second cell |
||||
|
||||
|
||||
## Ressources: |
||||
|
||||
- https://www.python.org/ |
||||
- https://docs.conda.io/ |
||||
- https://jupyter.org/ |
||||
- https://numpy.org/ |
||||
- https://towardsdatascience.com/jypyter-notebook-shortcuts-bf0101a98330 |
||||
- https://odsc.medium.com/why-you-should-be-using-jupyter-notebooks-ea2e568c59f2 |
@ -1,19 +0,0 @@
|
||||
##### This exercise is validated if the your_numpy_array is a NumPy array. It can be checked with `type(your_numpy_array)` that should be equal to `numpy.ndarray`. And if the type of is element are as follow. |
||||
|
||||
##### Try and run the following code. |
||||
|
||||
```python |
||||
for i in your_np_array: |
||||
print(type(i)) |
||||
|
||||
<class 'int'> |
||||
<class 'float'> |
||||
<class 'str'> |
||||
<class 'dict'> |
||||
<class 'list'> |
||||
<class 'tuple'> |
||||
<class 'set'> |
||||
<class 'bool'> |
||||
``` |
||||
|
||||
###### Does it display the right types as above? |
@ -1,21 +0,0 @@
|
||||
# Exercise 1 Your first NumPy array |
||||
|
||||
The goal of this exercise is to use many Python data types in **NumPy** arrays. **NumPy** arrays are intensively used in **NumPy** and **Pandas**. They are flexible and allow to use optimized **NumPy** underlying functions. |
||||
|
||||
1. Create a NumPy array that contains: an integer, a float, a string, a dictionary, a list, a tuple, a set and a boolean. |
||||
|
||||
The expected output is: |
||||
|
||||
```python |
||||
for i in your_np_array: |
||||
print(type(i)) |
||||
|
||||
<class 'int'> |
||||
<class 'float'> |
||||
<class 'str'> |
||||
<class 'dict'> |
||||
<class 'list'> |
||||
<class 'tuple'> |
||||
<class 'set'> |
||||
<class 'bool'> |
||||
``` |
@ -1,3 +0,0 @@
|
||||
##### The exercice is validated is all questions of the exercice are validated |
||||
##### The question 1 is validated is the solution uses `np.zeros` and if the shape of the array is `(300,)` |
||||
##### The question 2 is validated if the solution uses `reshape` and the shape of the array is `(3, 100)` |
@ -1,6 +0,0 @@
|
||||
# Exercise 2 Zeros |
||||
|
||||
The goal of this exercise is to learn to create a NumPy array with 0s. |
||||
|
||||
1. Create a NumPy array of dimension **300** with zeros without filling it manually |
||||
2. Reshape it to **(3,100)** |
@ -1,15 +0,0 @@
|
||||
##### The exercice is validated is all questions of the exercice are validated |
||||
|
||||
|
||||
##### The question 1 is validated if the solution doesn't involve a for loop or writing all integers from 1 to 100 and if the array is: `np.array([1,...,100])`. The list from 1 to 100 can be generated with an iterator: `range`. |
||||
|
||||
##### The question 2 is validated if the solution is: `integers[::2]` |
||||
|
||||
##### The question 3 is validated if the solution is: `integers[::-2]` |
||||
|
||||
##### The question 4 is validated if the array is: `np.array([0, 1,0,3,4,0,...,0,99,100])`. There are at least two ways to get this results without for loop. The first one uses `integers[1::3] = 0` and the second involves creating a boolean array that indexes the array: |
||||
|
||||
```python |
||||
mask = (integers+1)%3 == 0 |
||||
integers[mask] = 0 |
||||
``` |
@ -1,9 +0,0 @@
|
||||
# Exercise 3 Slicing |
||||
|
||||
The goal of this exercise is to learn NumPy indexing/slicing. It allows to access values of the NumPy array efficiently and without a for loop. |
||||
|
||||
1. Create a NumPy array of dimension 1 that contains all integers from 1 to 100 ordered. |
||||
2. Without using a for loop and using the array created in Q1, create an array that contain all odd integers. The expected output is: `np.array([1,3,...,99])`. *Hint*: it takes one line |
||||
3. Without using a for loop and using the array created in Q1, create an array that contain all even integers reversed. The expected output is: `np.array([100,98,...,2])`. *Hint*: it takes one line |
||||
|
||||
4. Using array of Q1, set the value of every 3 elements of the list (starting with the second) to 0. The expected output is: `np.array([[1,0,3,4,0,...,0,99,100]])` |
@ -1,40 +0,0 @@
|
||||
##### The exercice is validated is all questions of the exercice are validated |
||||
|
||||
##### For this exercise, as the results may change depending on the version of the package or the OS, I give the code to correct the exercise. If the code is correct and the output is not the same as mine, it is accepted. |
||||
|
||||
##### The question 1 is validated if the solution is: `np.random.seed(888)` |
||||
|
||||
##### The question 2 is validated if the solution is: `np.random.randn(100)`. The value of the first element is `0.17620087373662233`. |
||||
|
||||
##### The question 3 is validated if the solution is: `np.random.randint(1,11,(8,8))`. |
||||
|
||||
```console |
||||
Given the NumPy version and the seed, you should have this output: |
||||
|
||||
array([[ 7, 4, 8, 10, 2, 1, 1, 10], |
||||
[ 4, 1, 7, 4, 3, 5, 2, 8], |
||||
[ 3, 9, 7, 4, 9, 6, 10, 5], |
||||
[ 7, 10, 3, 10, 2, 1, 3, 7], |
||||
[ 3, 2, 3, 2, 10, 9, 5, 4], |
||||
[ 4, 1, 9, 7, 1, 4, 3, 5], |
||||
[ 3, 2, 10, 8, 6, 3, 9, 4], |
||||
[ 4, 4, 9, 2, 8, 5, 9, 5]]) |
||||
``` |
||||
|
||||
##### The question 4 is validated if the solution is: `np.random.randint(1,18,(4,2,5))`. |
||||
|
||||
```console |
||||
Given the NumPy version and the seed, you should have this output: |
||||
|
||||
array([[[14, 16, 8, 15, 14], |
||||
[17, 13, 1, 4, 17]], |
||||
|
||||
[[ 7, 15, 2, 8, 3], |
||||
[ 9, 4, 13, 9, 15]], |
||||
|
||||
[[ 5, 11, 11, 14, 10], |
||||
[ 2, 1, 15, 3, 3]], |
||||
|
||||
[[ 3, 10, 5, 16, 13], |
||||
[17, 12, 9, 7, 16]]]) |
||||
``` |
@ -1,17 +0,0 @@
|
||||
# Exercise 4 Random |
||||
|
||||
The goal of this exercise is to learn to generate random data. |
||||
In Data Science it is extremely useful to generate random data for many reasons: |
||||
Lack of real data, create a random benchmark, use varied data sets. |
||||
NumPy proposes a lot of options to generate random data. In statistics, assumptions are made on the distribution the data is from. All data distribution that can be generated randomly are described in the documentation. In this exercise we will focus on two distributions: |
||||
|
||||
- Uniform: For example, if your goal is to generate a random number from 1 to 100 and that the probability that all the numbers is equal you'll need the uniform distribution. NumPy provides `randint` and `uniform` to generate uniform distribution |
||||
|
||||
- Normal: The normal distribution is the most important probability distribution in statistics because it fits many natural phenomena.For example, if you need to generate a data sample that represents **Heights of 14 Year Old Girls** it can be done using the normal distribution. In that case, we need two parameters: the mean (1m51) and the standard deviation (0.0741m). NumPy provides `randn` to generate normal distribution (among other) |
||||
|
||||
https://numpy.org/doc/stable/reference/random/generator.html |
||||
|
||||
1. Set the seed to 888 |
||||
2. Generate a **one-dimensional** array of size 100 with a normal distribution |
||||
3. Generate a **two-dimensional** array of size 8,8 with random integers from 1 to 10 - both included (same probability for each integer) |
||||
4. Generate a **three-dimensional** of size 4,2,5 array with random integers from 1 to 17 - both included (same probability for each integer) |
@ -1,19 +0,0 @@
|
||||
##### The exercice is validated is all questions of the exercice are validated |
||||
|
||||
##### The question 1 is validated if the generated array is based on an iterator as `range` or `np.arange`. Check that 50 is part of the array. |
||||
|
||||
##### The question 2 is validated if the generated array is based on an iterator as `range` or `np.arange`. Check that 100 is part of the array. |
||||
|
||||
##### The question 3 is validated if you concatenated this way `np.concatenate(array1,array2)`. |
||||
|
||||
##### The question 4 is validated if the result is: |
||||
|
||||
```console |
||||
array([[ 1, ... , 10], |
||||
... |
||||
[ 91, ... , 100]]) |
||||
``` |
||||
|
||||
The easiest way is to use `array.reshape(10,10)`. |
||||
|
||||
https://jakevdp.github.io/PythonDataScienceHandbook/ (section: The Basics of NumPy Arrays) |
@ -1,17 +0,0 @@
|
||||
# Exercise 5: Split, concatenate, reshape arrays |
||||
|
||||
The goal of this exercise is to learn to concatenate and reshape arrays. |
||||
|
||||
1. Generate an array with integers from 1 to 50: `array([1,...,50])` |
||||
|
||||
2. Generate an array with integers from 51 to 100: `array([51,...,100])` |
||||
|
||||
3. Using `np.concatenate`, concatenate the two arrays into: `array([1,...,100])` |
||||
|
||||
4. Reshape the previous array into: |
||||
|
||||
```console |
||||
array([[ 1, ... , 10], |
||||
... |
||||
[ 91, ... , 100]]) |
||||
``` |
@ -1,28 +0,0 @@
|
||||
##### The exercice is validated is all questions of the exercice are validated |
||||
|
||||
##### The question 1 is validated if the output is the same as: |
||||
`np.ones([9,9], dtype=np.int8)` |
||||
|
||||
##### The question 2 is validated if the output is |
||||
|
||||
```console |
||||
array([[1, 1, 1, 1, 1, 1, 1, 1, 1], |
||||
[1, 0, 0, 0, 0, 0, 0, 0, 1], |
||||
[1, 0, 1, 1, 1, 1, 1, 0, 1], |
||||
[1, 0, 1, 0, 0, 0, 1, 0, 1], |
||||
[1, 0, 1, 0, 1, 0, 1, 0, 1], |
||||
[1, 0, 1, 0, 0, 0, 1, 0, 1], |
||||
[1, 0, 1, 1, 1, 1, 1, 0, 1], |
||||
[1, 0, 0, 0, 0, 0, 0, 0, 1], |
||||
[1, 1, 1, 1, 1, 1, 1, 1, 1]], dtype=int8) |
||||
``` |
||||
|
||||
##### The solution of question 2 is not accepted if the values of the array have been changed one by one manually. The usage of the for loop is not allowed neither. |
||||
Here is an example of a possible solution: |
||||
|
||||
```console |
||||
x[1:8,1:8] = 0 |
||||
x[2:7,2:7] = 1 |
||||
x[3:6,3:6] = 0 |
||||
x[4,4] = 1 |
||||
``` |
@ -1,20 +0,0 @@
|
||||
# Exercise 6: Broadcasting and Slicing |
||||
|
||||
The goal of this exercise is to learn to access values of n-dimensional arrays efficiently. |
||||
|
||||
1. Create an 2-dimensional array size 9,9 of 1s. Each value has to be an `int8`. |
||||
2. Using **slicing**, output this array: |
||||
|
||||
```python |
||||
array([[1, 1, 1, 1, 1, 1, 1, 1, 1], |
||||
[1, 0, 0, 0, 0, 0, 0, 0, 1], |
||||
[1, 0, 1, 1, 1, 1, 1, 0, 1], |
||||
[1, 0, 1, 0, 0, 0, 1, 0, 1], |
||||
[1, 0, 1, 0, 1, 0, 1, 0, 1], |
||||
[1, 0, 1, 0, 0, 0, 1, 0, 1], |
||||
[1, 0, 1, 1, 1, 1, 1, 0, 1], |
||||
[1, 0, 0, 0, 0, 0, 0, 0, 1], |
||||
[1, 1, 1, 1, 1, 1, 1, 1, 1]], dtype=int8) |
||||
``` |
||||
|
||||
https://jakevdp.github.io/PythonDataScienceHandbook/ (section: Computation on Arrays: Broadcasting) |
@ -1,32 +0,0 @@
|
||||
##### The exercice is validated is all questions of the exercice are validated |
||||
|
||||
##### This question is validated if, without having used a for loop or having filled the array manually, the output is: |
||||
|
||||
```console |
||||
[[ 7. 1. 7.] |
||||
[nan 2. 2.] |
||||
[nan 8. 8.] |
||||
[ 9. 3. 9.] |
||||
[ 8. 9. 8.] |
||||
[nan 2. 2.] |
||||
[ 8. 2. 8.] |
||||
[nan 6. 6.] |
||||
[ 9. 2. 9.] |
||||
[ 8. 5. 8.]] |
||||
``` |
||||
|
||||
There are two steps in this exercise: |
||||
|
||||
- Create the vector that contains the grade of the first exam if available or the second. This can be done using `np.where`: |
||||
|
||||
```python |
||||
np.where(np.isnan(grades[:, 0]), grades[:, 1], grades[:, 0]) |
||||
``` |
||||
|
||||
- Add this vector as third column of the array. Here are two ways: |
||||
|
||||
```python |
||||
np.insert(arr = grades, values = new_vector, axis = 1, obj = 2) |
||||
|
||||
np.hstack((grades, new_vector[:, None])) |
||||
``` |
@ -1,18 +0,0 @@
|
||||
# Exercise 7: NaN |
||||
|
||||
The goal of this exercise is to learn to deal with missing data in NumPy and to manipulate NumPy arrays. |
||||
|
||||
Let us consider a 2-dimensional array that contains the grades at the past two exams. Some of the students missed the first exam. As the grade is missing it has been replaced with a `NaN`. |
||||
|
||||
1. Using `np.where` create a third column that is equal to the grade of the first exam if it exists and the second else. Add the column as the third column of the array. |
||||
|
||||
**Using a for loop or if/else statement is not allowed in this exercise.** |
||||
|
||||
```python |
||||
import numpy as np |
||||
|
||||
generator = np.random.default_rng(123) |
||||
grades = np.round(generator.uniform(low = 0.0, high = 10.0, size = (10, 2))) |
||||
grades[[1,2,5,7], [0,0,0,0]] = np.nan |
||||
print(grades) |
||||
``` |
@ -1,52 +0,0 @@
|
||||
1. This question is validated if the text file has successfully been loaded in a NumPy array with |
||||
`genfromtxt('winequality-red.csv', delimiter=',')` and the reduced arrays weights **76800 bytes** |
||||
|
||||
2. This question is validated if the output is |
||||
|
||||
```python |
||||
array([[ 7.4 , 0.7 , 0. , 1.9 , 0.076 , 11. , 34. , |
||||
0.9978, 3.51 , 0.56 , 9.4 , 5. ], |
||||
[ 7.4 , 0.66 , 0. , 1.8 , 0.075 , 13. , 40. , |
||||
0.9978, 3.51 , 0.56 , 9.4 , 5. ], |
||||
[ 6.7 , 0.58 , 0.08 , 1.8 , 0.097 , 15. , 65. , |
||||
0.9959, 3.28 , 0.54 , 9.2 , 5. ]]) |
||||
``` |
||||
|
||||
This slicing gives the answer `my_data[[1,6,11],:]`. |
||||
|
||||
3. This question is validated if the answer if False. There many ways to get the answer: find the maximum or check values greater than 20. |
||||
|
||||
4. This question is validated if the answer is 10.422983114446529. |
||||
|
||||
5. This question is validated if the answers is: |
||||
|
||||
```console |
||||
pH stats |
||||
25 percentile: 3.21 |
||||
50 percentile: 3.31 |
||||
75 percentile: 3.4 |
||||
mean: 3.3111131957473416 |
||||
min: 2.74 |
||||
max: 4.01 |
||||
``` |
||||
|
||||
> *Note: Using `percentile` or `median` may give different results depending on the duplicate values in the column. If you do not have my results please use `percentile`.* |
||||
|
||||
6. This question is validated if the answer is ~`5.2`. The first step is to get the percentile 20% of the column `sulphates`, then create a boolean array that contains `True` of the value is smaller than the percentile 20%, then select this rows with the column quality and compute the `mean`. |
||||
|
||||
7. This question is validated if the output for the best wines is: |
||||
|
||||
```python |
||||
array([ 8.56666667, 0.42333333, 0.39111111, 2.57777778, 0.06844444, |
||||
13.27777778, 33.44444444, 0.99521222, 3.26722222, 0.76777778, |
||||
12.09444444, 8. ]) |
||||
``` |
||||
|
||||
And the output for the bad wines is: |
||||
|
||||
```python |
||||
array([ 8.36 , 0.8845 , 0.171 , 2.635 , 0.1225 , 11. , |
||||
24.9 , 0.997464, 3.398 , 0.57 , 9.955 , 3. ]) |
||||
``` |
||||
|
||||
This can be done in three steps: Get the max, create a boolean mask that indicates rows with max quality, use this mask to subset the rows with the best quality and compute the mean on the axis 0. |
@ -1,72 +0,0 @@
|
||||
Citation Request: |
||||
This dataset is public available for research. The details are described in [Cortez et al., 2009]. |
||||
Please include this citation if you plan to use this database: |
||||
|
||||
P. Cortez, A. Cerdeira, F. Almeida, T. Matos and J. Reis. |
||||
Modeling wine preferences by data mining from physicochemical properties. |
||||
In Decision Support Systems, Elsevier, 47(4):547-553. ISSN: 0167-9236. |
||||
|
||||
Available at: [@Elsevier] http://dx.doi.org/10.1016/j.dss.2009.05.016 |
||||
[Pre-press (pdf)] http://www3.dsi.uminho.pt/pcortez/winequality09.pdf |
||||
[bib] http://www3.dsi.uminho.pt/pcortez/dss09.bib |
||||
|
||||
1. Title: Wine Quality |
||||
|
||||
2. Sources |
||||
Created by: Paulo Cortez (Univ. Minho), Antonio Cerdeira, Fernando Almeida, Telmo Matos and Jose Reis (CVRVV) @ 2009 |
||||
|
||||
3. Past Usage: |
||||
|
||||
P. Cortez, A. Cerdeira, F. Almeida, T. Matos and J. Reis. |
||||
Modeling wine preferences by data mining from physicochemical properties. |
||||
In Decision Support Systems, Elsevier, 47(4):547-553. ISSN: 0167-9236. |
||||
|
||||
In the above reference, two datasets were created, using red and white wine samples. |
||||
The inputs include objective tests (e.g. PH values) and the output is based on sensory data |
||||
(median of at least 3 evaluations made by wine experts). Each expert graded the wine quality |
||||
between 0 (very bad) and 10 (very excellent). Several data mining methods were applied to model |
||||
these datasets under a regression approach. The support vector machine model achieved the |
||||
best results. Several metrics were computed: MAD, confusion matrix for a fixed error tolerance (T), |
||||
etc. Also, we plot the relative importances of the input variables (as measured by a sensitivity |
||||
analysis procedure). |
||||
|
||||
4. Relevant Information: |
||||
|
||||
The two datasets are related to red and white variants of the Portuguese "Vinho Verde" wine. |
||||
For more details, consult: http://www.vinhoverde.pt/en/ or the reference [Cortez et al., 2009]. |
||||
Due to privacy and logistic issues, only physicochemical (inputs) and sensory (the output) variables |
||||
are available (e.g. there is no data about grape types, wine brand, wine selling price, etc.). |
||||
|
||||
These datasets can be viewed as classification or regression tasks. |
||||
The classes are ordered and not balanced (e.g. there are munch more normal wines than |
||||
excellent or poor ones). Outlier detection algorithms could be used to detect the few excellent |
||||
or poor wines. Also, we are not sure if all input variables are relevant. So |
||||
it could be interesting to test feature selection methods. |
||||
|
||||
5. Number of Instances: red wine - 1599; white wine - 4898. |
||||
|
||||
6. Number of Attributes: 11 + output attribute |
||||
|
||||
Note: several of the attributes may be correlated, thus it makes sense to apply some sort of |
||||
feature selection. |
||||
|
||||
7. Attribute information: |
||||
|
||||
For more information, read [Cortez et al., 2009]. |
||||
|
||||
Input variables (based on physicochemical tests): |
||||
1 - fixed acidity |
||||
2 - volatile acidity |
||||
3 - citric acid |
||||
4 - residual sugar |
||||
5 - chlorides |
||||
6 - free sulfur dioxide |
||||
7 - total sulfur dioxide |
||||
8 - density |
||||
9 - pH |
||||
10 - sulphates |
||||
11 - alcohol |
||||
Output variable (based on sensory data): |
||||
12 - quality (score between 0 and 10) |
||||
|
||||
8. Missing Attribute Values: None |
@ -1,24 +0,0 @@
|
||||
|
||||
# Exercise 8: Wine |
||||
|
||||
The goal of this exercise is to learn to perform a basic data analysis on real data using NumPy. |
||||
|
||||
The data set that will be used for this exercise is the red wine data set. |
||||
|
||||
https://archive.ics.uci.edu/ml/datasets/wine+quality |
||||
|
||||
How to tell if a given 2D array has null columns? |
||||
|
||||
1. Using `genfromtxt` load the data and reduce the size of the numpy array by optimizing the types. The sum of absolute differences between the original data set and the "memory" optimized one has to be smaller than 1.10**-3. I suggest to use `np.float32`. Check that the numpy array weights **76800 bytes**. |
||||
|
||||
2. Print 2nd, 7th and 12th rows as a two dimensional array |
||||
|
||||
3. Is there any wine with a percentage of alcohol greater than 20% ? Return True or False |
||||
|
||||
4. What is the average % of alcohol on all wines in the data set ? If needed, drop `np.nan` values |
||||
|
||||
5. Compute the minimum, the maximum, the 25th percentile, the 50th percentile, the 75th percentile, the median (50th percentile) of the pH |
||||
|
||||
6. Compute the average quality of the wines having the 20% least sulphates |
||||
|
||||
7. Compute the mean of all variables for wines having the best quality. Same question for the wines having the worst quality |
@ -1,6 +0,0 @@
|
||||
This exercise is validated if the output is: |
||||
|
||||
```console |
||||
[[0 3 1 2 4] |
||||
[7 6 8 9 5]] |
||||
``` |
@ -1,10 +0,0 @@
|
||||
nan -9.480000000000000426e+00 1.415000000000000036e+01 1.126999999999999957e+01 -5.650000000000000355e+00 3.330000000000000071e+00 1.094999999999999929e+01 -2.149999999999999911e+00 5.339999999999999858e+00 -2.830000000000000071e+00 |
||||
9.480000000000000426e+00 nan 4.860000000000000320e+00 -8.609999999999999432e+00 7.820000000000000284e+00 -1.128999999999999915e+01 1.324000000000000021e+01 4.919999999999999929e+00 2.859999999999999876e+00 9.039999999999999147e+00 |
||||
-1.415000000000000036e+01 -1.126999999999999957e+01 nan 1.227999999999999936e+01 -2.410000000000000142e+00 6.040000000000000036e+00 -5.160000000000000142e+00 -3.870000000000000107e+00 -1.281000000000000050e+01 1.790000000000000036e+00 |
||||
5.650000000000000355e+00 -3.330000000000000071e+00 -1.094999999999999929e+01 nan -1.364000000000000057e+01 0.000000000000000000e+00 2.240000000000000213e+00 -3.609999999999999876e+00 -7.730000000000000426e+00 8.000000000000000167e-02 |
||||
2.149999999999999911e+00 -5.339999999999999858e+00 2.830000000000000071e+00 -4.860000000000000320e+00 nan -8.800000000000000044e-01 -8.570000000000000284e+00 2.560000000000000053e+00 -7.030000000000000249e+00 -6.330000000000000071e+00 |
||||
8.609999999999999432e+00 -7.820000000000000284e+00 1.128999999999999915e+01 -1.324000000000000021e+01 -4.919999999999999929e+00 nan -1.296000000000000085e+01 -1.282000000000000028e+01 -1.403999999999999915e+01 1.456000000000000050e+01 |
||||
-2.859999999999999876e+00 -9.039999999999999147e+00 -1.227999999999999936e+01 2.410000000000000142e+00 -6.040000000000000036e+00 5.160000000000000142e+00 nan -1.091000000000000014e+01 -1.443999999999999950e+01 -1.372000000000000064e+01 |
||||
3.870000000000000107e+00 1.281000000000000050e+01 -1.790000000000000036e+00 1.364000000000000057e+01 -0.000000000000000000e+00 -2.240000000000000213e+00 3.609999999999999876e+00 nan 1.053999999999999915e+01 -1.417999999999999972e+01 |
||||
7.730000000000000426e+00 -8.000000000000000167e-02 8.800000000000000044e-01 8.570000000000000284e+00 -2.560000000000000053e+00 7.030000000000000249e+00 6.330000000000000071e+00 1.296000000000000085e+01 nan -1.169999999999999929e+01 |
||||
1.282000000000000028e+01 1.403999999999999915e+01 -1.456000000000000050e+01 1.091000000000000014e+01 1.443999999999999950e+01 1.372000000000000064e+01 -1.053999999999999915e+01 1.417999999999999972e+01 1.169999999999999929e+01 nan |
@ -1,26 +0,0 @@
|
||||
## Exercise 9 Football tournament |
||||
|
||||
The goal of this exercise is to learn to use permutations, complex |
||||
|
||||
A Football tournament is organized in your city. There are 10 teams and the director of the tournaments wants you to create a first round as exciting as possible. To do so, you are allowed to choose the pairs. As a former data scientist, you implemented a model based on teams' current season performance. This models predicts the score difference between two teams. You used this algorithm to predict the score difference for every possible pair. |
||||
The matrix returned is a 2-dimensional array that contains in (i,j) the score difference between team i and j. The matrix is in `model_forecasts.txt`. |
||||
|
||||
Using this output, what are the pairs that will give the most interesting matches ? |
||||
|
||||
If a team wins 7-1 the match is obviously less exciting than a match where the winner wins 2-1. |
||||
The criteria that corresponds to **the pairs that will give the most interesting matches** is **the pairs that minimize the sum of squared differences** |
||||
|
||||
The expected output is: |
||||
|
||||
```console |
||||
[[m1_t1 m2_t1 m3_t1 m4_t1 m5_t1] |
||||
[m1_t2 m2_t2 m3_t2 m4_t2 m5_t2]] |
||||
|
||||
``` |
||||
|
||||
- m1_t1 stands for match1_team1 |
||||
- m1_t1 plays against m1_t2 ... |
||||
|
||||
**Usage of for loop is not allowed, you may need to use the library** `itertools` **to create permutations** |
||||
|
||||
https://docs.python.org/3.9/library/itertools.html |
@ -1,31 +0,0 @@
|
||||
# W1D01 Piscine AI - Data Science |
||||
|
||||
## NumPy |
||||
The goal of this day is to understand practical usage of **NumPy**. **NumPy** is a commonly used Python data analysis package. By using **NumPy**, you can speed up your workflow, and interface with other packages in the Python ecosystem, like scikit-learn, that use **NumPy** under the hood. **NumPy** was originally developed in the mid 2000s, and arose from an even older package called Numeric. This longevity means that almost every data analysis or machine learning package for Python leverages **NumPy** in some way. |
||||
|
||||
## Exercises of the day |
||||
|
||||
- Exercise 0 Environment and libraries |
||||
- Exercise 1 Your first NumPy array |
||||
- Exercise 2 Zeros |
||||
- Exercise 3 Slicing |
||||
- Exercise 4 Random |
||||
- Exercise 5 Split, concatenate, reshape arrays |
||||
- Exercise 6 Broadcasting and Slicing |
||||
- Exercise 7 NaN |
||||
- Exercise 8 Wine |
||||
- Exercise 9 Football tournament |
||||
|
||||
## Virtual Environment |
||||
- Python 3.x |
||||
- NumPy |
||||
- Jupyter or JupyterLab |
||||
|
||||
*Version of NumPy I used to do the exercises: 1.18.1*. |
||||
I suggest to use the most recent one. |
||||
|
||||
## Ressources |
||||
|
||||
- https://medium.com/fintechexplained/why-should-we-use-NumPy-c14a4fb03ee9 |
||||
- https://numpy.org/doc/ |
||||
- https://jakevdp.github.io/PythonDataScienceHandbook/ |
@ -1,9 +0,0 @@
|
||||
##### The exercice is validated is all questions of the exercice are validated |
||||
|
||||
##### Activate the virtual environment. If you used `conda` run `conda activate your_env` |
||||
|
||||
##### Run `python --version` |
||||
|
||||
###### Does it print `Python 3.x`? x >= 8 |
||||
|
||||
##### Does `import jupyter`, `import numpy` and `import pandas` run without any error ? |
@ -1,64 +0,0 @@
|
||||
# W1D02 Piscine AI - Data Science |
||||
|
||||
## Pandas |
||||
|
||||
The goal of this day is to understand practical usage of **Pandas**. |
||||
As **Pandas** in intensively used in Data Science, other days of the piscine will be dedicated to it. |
||||
|
||||
Not only is the **Pandas** library a central component of the data science toolkit but it is used in conjunction with other libraries in that collection. |
||||
|
||||
**Pandas** is built on top of the NumPy package, meaning a lot of the structure of NumPy is used or replicated in **Pandas**. Data in **Pandas** is often used to feed statistical analysis in SciPy, plotting functions from Matplotlib, and machine learning algorithms in Scikit-learn. |
||||
|
||||
Most of the topics we will cover today are explained and describes with examples in the first resource. The number of exercises is low on purpose: Take the time to understand the chapter 5 of the resource, even if there are 40 pages. |
||||
|
||||
## Exercises of the day |
||||
|
||||
- Exercice 0 Environment and libraries |
||||
- Exercise 1 Your first DataFrame |
||||
- Exercise 2 Electric power consumption |
||||
- Exercise 3 E-commerce purchases |
||||
- Exercise 4 Handling missing values |
||||
|
||||
## Virtual Environment |
||||
- Python 3.x |
||||
- NumPy |
||||
- Pandas |
||||
- Jupyter or JupyterLab |
||||
|
||||
*Version of Pandas I used to do the exercises: 1.0.1*. |
||||
I suggest to use the most recent one. |
||||
|
||||
## Resources |
||||
|
||||
- If I had to give you one resource it would be this one: |
||||
|
||||
https://bedford-computing.co.uk/learning/wp-content/uploads/2015/10/Python-for-Data-Analysis.pdf |
||||
|
||||
It contains ALL you need to know about Pandas. |
||||
|
||||
- Pandas documentation: |
||||
|
||||
- https://pandas.pydata.org/docs/ |
||||
|
||||
- https://jakevdp.github.io/PythonDataScienceHandbook/ |
||||
|
||||
- https://pandas.pydata.org/Pandas_Cheat_Sheet.pdf |
||||
|
||||
- https://www.learndatasci.com/tutorials/python-pandas-tutorial-complete-introduction-for-beginners/ |
||||
|
||||
- https://jakevdp.github.io/PythonDataScienceHandbook/03.04-missing-values.html |
||||
|
||||
# Exercise 0 Environment and libraries |
||||
|
||||
The goal of this exercise is to set up the Python work environment with the required libraries. |
||||
|
||||
**Note:** For each quest, your first exercice will be to set up the virtual environment with the required libraries. |
||||
|
||||
I recommend to use: |
||||
|
||||
- the **last stable versions** of Python. |
||||
- the virtual environment you're the most confortable with. `virtualenv` and `conda` are the most used in Data Science. |
||||
- one of the most recents versions of the libraries required |
||||
|
||||
1. Create a virtual environment named `ex00`, with a version of Python >= `3.8`, with the following libraries: `pandas`, `numpy` and `jupyter`. |
||||
|
@ -1,17 +0,0 @@
|
||||
##### The exercice is validated is all questions of the exercice are validated |
||||
|
||||
##### The solution of question 1 is accepted if the DataFrame created is the same as the "model" DataFrame. Check that the index is not 1,2,3,4,5. |
||||
|
||||
##### The solution of question 2 is accepted if the types you get for the columns are as below and if if the types of the first value of the columns are as below |
||||
|
||||
```console |
||||
<class 'pandas.core.series.Series'> |
||||
<class 'pandas.core.series.Series'> |
||||
<class 'pandas.core.series.Series'> |
||||
``` |
||||
|
||||
```console |
||||
<class 'str'> |
||||
<class 'list'> |
||||
<class 'float'> |
||||
``` |
@ -1,17 +0,0 @@
|
||||
# Exercice 1 |
||||
|
||||
The goal of this exercise is to learn to create basic Pandas objects. |
||||
|
||||
1. Create a DataFrame as below this using two ways: |
||||
- From a NumPy array |
||||
- From a Pandas Series |
||||
|
||||
| | color | list | number | |
||||
|---:|:--------|:--------|---------:| |
||||
| 1 | Blue | [1, 2] | 1.1 | |
||||
| 3 | Red | [3, 4] | 2.2 | |
||||
| 5 | Pink | [5, 6] | 3.3 | |
||||
| 7 | Grey | [7, 8] | 4.4 | |
||||
| 9 | Black | [9, 10] | 5.5 | |
||||
|
||||
2. Print the types for every columns and the types of the first value of every columns |
@ -1,101 +0,0 @@
|
||||
##### The exercice is validated is all questions of the exercice are validated |
||||
|
||||
##### The solution of question 1 is accepted if you use `drop` with `axis=1`.`inplace=True` may be useful to avoid to affect the result to a variable. A solution that could be accepted too (even if it's not a solution I recommend is `del`. |
||||
|
||||
##### The solution of question 2 is accepted if the DataFrame returns the output below. If the type of the index is not `dtype='datetime64[ns]'` the solution is not accepted. I recommend to use `set_index` with `inplace=True` to do so. |
||||
|
||||
```python |
||||
Input: df.head().index |
||||
|
||||
Output: |
||||
|
||||
DatetimeIndex(['2006-12-16', '2006-12-16','2006-12-16', '2006-12-16','2006-12-16'], |
||||
dtype='datetime64[ns]', name='Date', freq=None) |
||||
``` |
||||
|
||||
##### The solution of question 3 is accepted if all the types are `float64` as below. The preferred solution is `pd.to_numeric` with `coerce=True`. |
||||
|
||||
```python |
||||
Input: df.dtypes |
||||
|
||||
Output: |
||||
|
||||
Global_active_power float64 |
||||
Global_reactive_power float64 |
||||
Voltage float64 |
||||
Global_intensity float64 |
||||
Sub_metering_1 float64 |
||||
dtype: object |
||||
|
||||
``` |
||||
|
||||
##### The solution of question 4 is accepted if you use `df.describe()`. |
||||
|
||||
##### The solution of question 5 is accepted if you used `dropna` and have the number of missing values equal to 0.You should have noticed that 25979 rows contain missing values (for a total of 129895). `df.isna().sum()` allows to check the number of missing values and `df.dropna()` with `inplace=True` allows to remove the rows with missing values. |
||||
|
||||
##### The solution of question 6 is accepted if one of the two approaches below were used: |
||||
|
||||
```python |
||||
#solution 1 |
||||
df.loc[:,'A'] = (df['A'] + 1) * 0.06 |
||||
|
||||
#solution 2 |
||||
df.loc[:,'A'] = df.loc[:,'A'].apply(lambda x: (x+1)*0.06) |
||||
|
||||
``` |
||||
|
||||
|
||||
You may wonder `df.loc[:,'A']` is required and if `df['A'] = ...` works too. **The answer is no**. This is important in Pandas. Depending on the version of Pandas, it may return a warning. The reason is that you are affecting a value to a **copy** of the DataFrame and not in the DataFrame. |
||||
More details: https://stackoverflow.com/questions/20625582/how-to-deal-with-settingwithcopywarning-in-pandas |
||||
|
||||
##### The solution of question 7 is accepted as long as the output of `print(filtered_df.head().to_markdown())` is as below and if the number of rows is equal to **449667**. |
||||
|
||||
| Date | Global_active_power | Global_reactive_power | |
||||
|:--------------------|----------------------:|------------------------:| |
||||
| 2008-12-27 00:00:00 | 0.996 | 0.066 | |
||||
| 2008-12-27 00:00:00 | 1.076 | 0.162 | |
||||
| 2008-12-27 00:00:00 | 1.064 | 0.172 | |
||||
| 2008-12-27 00:00:00 | 1.07 | 0.174 | |
||||
| 2008-12-27 00:00:00 | 0.804 | 0.184 | |
||||
|
||||
##### The solution of question 8 is accepted if the output is |
||||
|
||||
```console |
||||
Global_active_power 0.254 |
||||
Global_reactive_power 0.000 |
||||
Voltage 238.350 |
||||
Global_intensity 1.200 |
||||
Sub_metering_1 0.000 |
||||
Name: 2007-02-16 00:00:00, dtype: float64 |
||||
|
||||
``` |
||||
|
||||
##### The solution of question 9 if the output is `Timestamp('2009-02-22 00:00:00')` |
||||
|
||||
##### The solution of question 10 if the output of `print(sorted_df.tail().to_markdown())` is |
||||
|
||||
| Date | Global_active_power | Global_reactive_power | Voltage | |
||||
|:--------------------|----------------------:|------------------------:|----------:| |
||||
| 2008-08-28 00:00:00 | 0.076 | 0 | 234.88 | |
||||
| 2008-08-28 00:00:00 | 0.076 | 0 | 235.18 | |
||||
| 2008-08-28 00:00:00 | 0.076 | 0 | 235.4 | |
||||
| 2008-08-28 00:00:00 | 0.076 | 0 | 235.64 | |
||||
| 2008-12-08 00:00:00 | 0.076 | 0 | 236.5 | |
||||
|
||||
##### The solution of question 11 is accepted if the output is as below. The solution is based on `groupby` which creates groups based on the index `Date` and aggregates the groups using the `mean`. |
||||
|
||||
```console |
||||
Date |
||||
2006-12-16 3.053475 |
||||
2006-12-17 2.354486 |
||||
2006-12-18 1.530435 |
||||
2006-12-19 1.157079 |
||||
2006-12-20 1.545658 |
||||
... |
||||
2010-12-07 0.770538 |
||||
2010-12-08 0.367846 |
||||
2010-12-09 1.119508 |
||||
2010-12-10 1.097008 |
||||
2010-12-11 1.275571 |
||||
Name: Global_active_power, Length: 1433, dtype: float64 |
||||
``` |
@ -1 +0,0 @@
|
||||
Empty file. The original is too big to be pushed on Github. |
@ -1,24 +0,0 @@
|
||||
# Exercise 2 **Electric power consumption** |
||||
|
||||
The goal of this exercise is to learn to manipulate real data with Pandas. |
||||
|
||||
The data set used is **Individual household electric power consumption** |
||||
|
||||
1. Delete the columns `Time`, `Sub_metering_2` and `Sub_metering_3` |
||||
2. Set `Date` as index |
||||
3. Create a function that takes as input the DataFrame with the data set and returns a DataFrame with updated types: |
||||
|
||||
```python |
||||
def update_types(df): |
||||
#TODO |
||||
return df |
||||
``` |
||||
|
||||
4. Use `describe` to have an overview on the data set |
||||
5. Delete the rows with missing values |
||||
6. Modify `Sub_metering_1` by adding 1 to it and multiplying the total by 0.06. If x is a row the output is: (x+1)*0.06 |
||||
7. Select all the rows for which the Date is greater or equal than 2008-12-27 and `Voltage` is greater or equal than 242 |
||||
8. Print the 88888th row. |
||||
9. What is the date for which the `Global_active_power` is maximal ? |
||||
10. Sort the first three columns by descending order of `Global_active_power` and ascending order of `Voltage`. |
||||
11. Compute the daily average of `Global_active_power`. |
@ -1,49 +0,0 @@
|
||||
##### The exercice is validated is all questions of the exercice are validated |
||||
|
||||
##### To validate this exercise all answers should return the expected numerical value given in the correction AND uses Pandas. For example using NumPy to compute the mean doesn't respect the philosophy of the exercise which is to use Pandas. |
||||
|
||||
#### The solution of question 1 is accepted if it contains **10000 entries** and **14 columns**. There many solutions based on: shape, info, describe. |
||||
|
||||
##### The solution of question 2 is accepted if the answer is **50.34730200000025**. |
||||
|
||||
Even if `np.mean` gives the solution, `df['Purchase Price'].mean()` is preferred |
||||
|
||||
##### The solution of question 3 is accepted if the min is `0`and the max is `99.989999999999995` |
||||
|
||||
|
||||
##### The solution of question 4 is accepted if the answer is **1098** |
||||
|
||||
##### The solution of question 5 is accepted if the answer is **30** |
||||
|
||||
##### The solution of question 6 is accepted if the are `4932` people that made the purchase during the `AM` and `5068` people that made the purchase during `PM`. There many ways to the solution but the goal of this question was to make you use `value_counts` |
||||
|
||||
##### The solution of question 7 is accepted if the answer is as below. There many ways to the solution but the goal of this question was to make you use `value_counts` |
||||
|
||||
Interior and spatial designer 31 |
||||
|
||||
Lawyer 30 |
||||
|
||||
Social researcher 28 |
||||
|
||||
Purchasing manager 27 |
||||
|
||||
Designer, jewellery 27 |
||||
|
||||
|
||||
8. ##### The solution of question 8 is accepted if the purchase price is **75.1** |
||||
|
||||
|
||||
##### The solution of question 9 is accepted if the email adress is **bondellen@williams-garza.com** |
||||
|
||||
##### The solution of question 10 is accepted if the answer is **39**. The prefered solution is based on this: `df[(df['A'] == X) & (df['B'] > Y)]` |
||||
|
||||
|
||||
##### The solution of question 11 is accepted if the answer is **1033**. The preferred solution is based on the usage of `apply` on a `lambda` function that slices the string that contains the expiration date. |
||||
|
||||
##### The solution of question 12 is accepted if the answer is as below. The preferred solution is based on the usage of `apply` on a `lambda` function that slices the string that contains the email. The `lambda` function uses `split` to split the string on `@`. Finally, `value_counts` is used to count the occurrences. |
||||
|
||||
- hotmail.com 1638 |
||||
- yahoo.com 1616 |
||||
- gmail.com 1605 |
||||
- smith.com 42 |
||||
- williams.com 37 |
@ -1,20 +0,0 @@
|
||||
# Exercice 3: E-commerce purchases<w> |
||||
|
||||
The goal of this exercise is to learn to manipulate real data with Pandas. This exercise is less guided since the exercise 2 should have given you a nice introduction. |
||||
|
||||
The data set used is **E-commerce purchases**. |
||||
|
||||
Questions: |
||||
|
||||
1. How many rows and columns are there? |
||||
2. What is the average Purchase Price? |
||||
3. What were the highest and lowest purchase prices? |
||||
4. How many people have English `'en'` as their Language of choice on the website? |
||||
5. How many people have the job title of `"Lawyer"` ? |
||||
6. How many people made the purchase during the `AM` and how many people made the purchase during `PM` ? |
||||
7. What are the 5 most common Job Titles? |
||||
8. Someone made a purchase that came from Lot: `"90 WT"` , what was the Purchase Price for this transaction? |
||||
9. What is the email of the person with the following Credit Card Number: `4926535242672853` |
||||
10. How many people have American Express as their Credit Card Provider and made a purchase above `$95` ? |
||||
11. How many people have a credit card that expires in `2025`? |
||||
12. What are the top 5 most popular email providers/hosts (e.g. gmail.com, yahoo.com, etc...) |
@ -1,32 +0,0 @@
|
||||
##### The exercice is validated is all questions of the exercice are validated (except the bonus question) |
||||
|
||||
##### The solution of question 1 is accepted if you have done these two steps in that order. First, convert the numerical columns to `float` and then fill the missing values. The first step may involve `pd.to_numeric(df.loc[:,col], errors='coerce')`. The second step is validated if you eliminated all missing values. However there are many possibilities to fill the missing values. Here is one of them: |
||||
|
||||
example: |
||||
|
||||
```python |
||||
df.fillna({0:df.sepal_length.mean(), |
||||
2:df.sepal_width.median(), |
||||
3:0, |
||||
4:0}) |
||||
``` |
||||
|
||||
##### The solution of question 2 is accepted if the solution is `df.loc[:,col].fillna(df[col].median())`. |
||||
|
||||
##### The solution of bonus question is accepted if you find out this answer: Once we filled the missing values as suggested in the first question, `df.describe()` returns this interesting summary. We notice that the mean is way higher than the median. It means that there are maybe some outliers in the data. The quantile 75 and the max confirm that: 75% of the flowers have a sepal length smaller than 6.4 cm, but the max is 6900 cm. If you check on the internet you realise this small flower can't be that big. The outliers have a major impact on the mean which equals to 56.9. Filling this value for the missing value is not correct since it doesn't correspond to the real size of this flower. That is why in that case the best strategy to fill the missing values is the median. The truth is that I modified the data set ! But real data sets ALWAYS contains outliers. Always think about the meaning of the data transformation ! If you fill the missing values by zero, it means that you consider that the length or width of some flowers may be 0. It doesn't make sense. |
||||
|
||||
|
||||
| | sepal_length | sepal_width | petal_length | petal_width | |
||||
|:------|---------------:|--------------:|---------------:|--------------:| |
||||
| count | 146 | 141 | 120 | 147 | |
||||
| mean | 56.9075 | 52.6255 | 15.5292 | 12.0265 | |
||||
| std | 572.222 | 417.127 | 127.46 | 131.873 | |
||||
| min | -4.4 | -3.6 | -4.8 | -2.5 | |
||||
| 25% | 5.1 | 2.8 | 2.725 | 0.3 | |
||||
| 50% | 5.75 | 3 | 4.5 | 1.3 | |
||||
| 75% | 6.4 | 3.3 | 5.1 | 1.8 | |
||||
| max | 6900 | 3809 | 1400 | 1600 | |
||||
|
||||
|
||||
|
||||
##### The solution of bonus question is accepted if you noticed that there are some negative values and the huge values, you will be a good data scientist. **YOU SHOULD ALWAYS TRY TO UNDERSTAND YOUR DATA**. Print the row with index 122 ;-) This week, we will have the opportunity to focus on the data pre-processing to understand how the outliers can be handled. |
|
@ -1,152 +0,0 @@
|
||||
sepal_length,sepal_width,petal_length,petal_width, flower |
||||
5.1,3.5,1.4,0.2,Iris-setosa |
||||
4.9,3.0,1.4,0.2,Iris-setosa |
||||
4.7,3.2,1.3,0.2,Iris-setosa |
||||
4.6,3.1,1.5,0.2,Iris-setosa |
||||
5.0,-3.6,-1.4,0.2,Iris-setosa |
||||
5.4,3.9,1.7,0.4,Iris-setosa |
||||
4.6,3.4,1.4,0.3,Iris-setosa |
||||
5.0,3.4,1.5,0.2,Iris-setosa |
||||
-4.4,2.9,1400,0.2,Iris-setosa |
||||
4.9,3.1,1.5,0.1,Iris-setosa |
||||
5.4,3.7,1.5,0.2,Iris-setosa |
||||
4.8,3.4,1.6,0.2,Iris-setosa |
||||
4.8,3.0,1.4,0.1,Iris-setosa |
||||
4.3,3.0,1.1,0.1,Iris-setosa |
||||
5.8,4.0,1.2,0.2,Iris-setosa |
||||
5.7,4.4,1500,0.4,Iris-setosa |
||||
5.4,3.9,1.3,0.4,Iris-setosa |
||||
5.1,3.5,1.4,0.3,Iris-setosa |
||||
5.7,3.8,1.7,0.3,Iris-setosa |
||||
5.1,3.8,1.5,0.3,Iris-setosa |
||||
5.4,3.4,-1.7,0.2,Iris-setosa |
||||
5.1,3.7,1.5,0.4,Iris-setosa |
||||
4.6,3.6,1.0,0.2,Iris-setosa |
||||
5.1,3.3,1.7,0.5,Iris-setosa |
||||
4.8,3.4,1.9,0.2,Iris-setosa |
||||
5.0,-3.0,1.6,0.2,Iris-setosa |
||||
5.0,3.4,1.6,0.4,Iris-setosa |
||||
5.2,3.5,1.5,0.2,Iris-setosa |
||||
5.2,3.4,1.4,0.2,Iris-setosa |
||||
4.7,3.2,1.6,0.2,Iris-setosa |
||||
4.8,3.1,1.6,0.2,Iris-setosa |
||||
5.4,3.4,1.5,0.4,Iris-setosa |
||||
5.2,4.1,1.5,0.1,Iris-setosa |
||||
5.5,4.2,1.4,0.2,Iris-setosa |
||||
4.9,3.1,1.5,0.1,Iris-setosa |
||||
5.0,3.2,1.2,0.2,Iris-setosa |
||||
5.5,3.5,1.3,0.2,Iris-setosa |
||||
4.9,,1.5,0.1,Iris-setosa |
||||
4.4,3.0,1.3,0.2,Iris-setosa |
||||
5.1,3.4,1.5,0.2,Iris-setosa |
||||
5.0,"3.5",1.3,0.3,Iris-setosa |
||||
4.5,2.3,1.3,0.3,Iris-setosa |
||||
4.4,3.2,1.3,0.2,Iris-setosa |
||||
5.0,3.5,1.6,0.6,Iris-setosa |
||||
5.1,3.8,1.9,0.4,Iris-setosa |
||||
4.8,3.0,1.4,0.3,Iris-setosa |
||||
5.1,3809,1.6,0.2,Iris-setosa |
||||
4.6,3.2,1.4,0.2,Iris-setosa |
||||
5.3,3.7,1.5,0.2,Iris-setosa |
||||
5.0,3.3,1.4,0.2,Iris-setosa |
||||
7.0,3.2,4.7,1.4,Iris-versicolor |
||||
6.4,3200,4.5,1.5,Iris-versicolor |
||||
6.9,3.1,4.9,1.5,Iris-versicolor |
||||
5.5,2.3,4.0,1.3,Iris-versicolor |
||||
6.5,2.8,4.6,1.5,Iris-versicolor |
||||
5.7,2.8,4.5,1.3,Iris-versicolor |
||||
6.3,3.3,4.7,1600,Iris-versicolor |
||||
4.9,2.4,3.3,1.0,Iris-versicolor |
||||
6.6,2.9,4.6,1.3,Iris-versicolor |
||||
5.2,2.7,3.9,,Iris-versicolor |
||||
5.0,2.0,3.5,1.0,Iris-versicolor |
||||
5.9,3.0,4.2,1.5,Iris-versicolor |
||||
6.0,2.2,4.0,1.0,Iris-versicolor |
||||
6.1,2.9,4.7,1.4,Iris-versicolor |
||||
5.6,2.9,3.6,1.3,Iris-versicolor |
||||
6.7,3.1,4.4,1.4,Iris-versicolor |
||||
5.6,3.0,4.5,1.5,Iris-versicolor |
||||
5.8,2.7,4.1,1.0,Iris-versicolor |
||||
6.2,2.2,4.5,1.5,Iris-versicolor |
||||
5.6,2.5,3.9,1.1,Iris-versicolor |
||||
5.9,3.2,4.8,1.8,Iris-versicolor |
||||
6.1,2.8,4.0,1.3,Iris-versicolor |
||||
6.3,2.5,4.9,1.5,Iris-versicolor |
||||
6.1,2.8,4.7,1.2,Iris-versicolor |
||||
6.4,2.9,4.3,1.3,Iris-versicolor |
||||
6.6,3.0,4.4,1.4,Iris-versicolor |
||||
6.8,2.8,4.8,1.4,Iris-versicolor |
||||
6.7,3.0,5.0,1.7,Iris-versicolor |
||||
6.0,2.9,4.5,1.5,Iris-versicolor |
||||
5.7,2.6,3.5,1.0,Iris-versicolor |
||||
5.5,2.4,3.8,1.1,Iris-versicolor |
||||
5.5,2.4,3.7,1.0,Iris-versicolor |
||||
5.8,2.7,3.9,1.2,Iris-versicolor |
||||
6.0,2.7,5.1,1.6,Iris-versicolor |
||||
5.4,3.0,4.5,1.5,Iris-versicolor |
||||
6.0,3.4,4.5,1.6,Iris-versicolor |
||||
6.7,3.1,4.7,1.5,Iris-versicolor |
||||
6.3,2.3,4.4,1.3,Iris-versicolor |
||||
5.6,3.0,4.1,1.3,Iris-versicolor |
||||
5.5,2.5,4.0,1.3,Iris-versicolor |
||||
5.5,2.6,4.4,1.2,Iris-versicolor |
||||
6.1,3.0,4.6,1.4,Iris-versicolor |
||||
5.8,2.6,4.0,1.2,Iris-versicolor |
||||
5.0,2.3,3.3,1.0,Iris-versicolor |
||||
5.6,2.7,4.2,1.3,Iris-versicolor |
||||
5.7,3.0,4.2,1.2,Iris-versicolor |
||||
5.7,2.9,4.2,1.3,Iris-versicolor |
||||
6.2,2.9,4.3,1.3,Iris-versicolor |
||||
5.1,2.5,3.0,1.1,Iris-versicolor |
||||
5.7,2.8,4.1,1.3,Iris-versicolor |
||||
6.3,3.3,6.0,2.5,Iris-virginica |
||||
5.8,2.7,5.1,1.9,Iris-virginica |
||||
7.1,3.0,5.9,2.1,Iris-virginica |
||||
6.3,2.9,5.6,1.8,Iris-virginica |
||||
6.5,3.0,5.8,2.2,Iris-virginica |
||||
7.6,3.0,6.6,2.1,Iris-virginica |
||||
4.9,2.5,4.5,1.7,Iris-virginica |
||||
7.3,2.9,6.3,1.8,Iris-virginica |
||||
6.7,2.5,5.8,1.8,Iris-virginica |
||||
7.2,3.6,6.1,2.5,Iris-virginica |
||||
6.5,3.2,5.1,2.0,Iris-virginica |
||||
6.4,2.7,5.3,1.9,Iris-virginica |
||||
6.8,3.0,5.5,2.1,Iris-virginica |
||||
5.7,2.5,5.0,2.0,Iris-virginica |
||||
5.8,2.8,5.1,2.4,Iris-virginica |
||||
6.4,3.2,5.3,2.3,Iris-virginica |
||||
6.5,3.0,5.5,1.8,Iris-virginica |
||||
7.7,3.8,6.7,2.2,Iris-virginica |
||||
7.7,2.6,6.9,2.3,Iris-virginica |
||||
6.0,2.2,5.0,1.5,Iris-virginica |
||||
6.9,3.2,5.7,2.3,Iris-virginica |
||||
5.6,2.8,4.9,2.0,Iris-virginica |
||||
7.7,2.8,6.7,2.0,Iris-virginica |
||||
6.3,2.7,4.9,1.8,Iris-virginica |
||||
6.7,3.3,5.7,2.1,Iris-virginica |
||||
7.2,3.2,6.0,1.8,Iris-virginica |
||||
6.2,2.8,-4.8,1.8,Iris-virginica |
||||
6.1,3.0,4.9,1.8,Iris-virginica |
||||
6.4,2.8,5.6,2.1,Iris-virginica |
||||
7.2,3.0,5.8,1.6,Iris-virginica |
||||
7.4,2.8,6.1,1.9,Iris-virginica |
||||
7.9,3.8,6.4,2.0,Iris-virginica |
||||
6.-4,2.8,5.6,2.2,Iris-virginica |
||||
6.3,2.8,"5.1",1.5,Iris-virginica |
||||
6.1,2.6,5.6,1.4,Iris-virginica |
||||
7.7,3.0,6.1,2.3,Iris-virginica |
||||
6.3,3.4,5.6,2.4,Iris-virginica |
||||
6.4,3.1,5.5,1.8,Iris-virginica |
||||
6.0,3.0,4.8,1.8,Iris-virginica |
||||
6900,3.1,5.4,2.1,Iris-virginica |
||||
6.7,3.1,5.6,2.4,Iris-virginica |
||||
6.9,3.1,5.1,2.3,Iris-virginica |
||||
580,2.7,5.1,1.9,Iris-virginica |
||||
6.8,3.2,5.9,2.3,Iris-virginica |
||||
6.7,3.3,5.7,-2.5,Iris-virginica |
||||
6.7,3.0,5.2,2.3,Iris-virginica |
||||
6.3,2.5,5.0,1.9,Iris-virginica |
||||
6.5,3.0,5.2,2.0,Iris-virginica |
||||
6.2,3.4,5.4,2.3,Iris-virginica |
||||
5.9,3.0,5.1,1.8,Iris-virginica |
||||
|
@ -1,26 +0,0 @@
|
||||
# Exercice 4 Handling missing values |
||||
|
||||
The goal of this exercise is to learn to handle missing values. In the previous exercise we used the first techniques: filter out the missing values. We were lucky because the proportion of missing values was low. But in some cases, dropping the missing values is not possible because the filtered data set would be too small. |
||||
|
||||
This article explains the different types of missing data and how they should be handled. |
||||
|
||||
https://towardsdatascience.com/data-cleaning-with-python-and-pandas-detecting-missing-values-3e9c6ebcf78b |
||||
|
||||
"**It’s important to understand these different types of missing data from a statistics point of view. The type of missing data will influence how you deal with filling in the missing values.**" |
||||
|
||||
- Preliminary: Drop the `flower` column |
||||
|
||||
1. Fill the missing values with a different "strategy" for each column: |
||||
|
||||
`sepal_length` -> `mean` |
||||
|
||||
`sepal_width` -> `median` |
||||
|
||||
`petal_length`, `petal_width` -> `0` |
||||
|
||||
2. Fill the missing values using the median of the associated column using `fillna`. |
||||
|
||||
|
||||
- Bonus questions: |
||||
- Filling the missing values by 0 or the mean of the associated column is common in Data Science. In that case, explain why filling the missing values with 0 or the mean is a bad idea. |
||||
- Find a special row ;-) |
@ -1,48 +0,0 @@
|
||||
# W1D02 Piscine AI - Data Science |
||||
|
||||
## Pandas |
||||
|
||||
The goal of this day is to understand practical usage of **Pandas**. |
||||
As **Pandas** in intensively used in Data Science, other days of the piscine will be dedicated to it. |
||||
|
||||
Not only is the **Pandas** library a central component of the data science toolkit but it is used in conjunction with other libraries in that collection. |
||||
|
||||
**Pandas** is built on top of the NumPy package, meaning a lot of the structure of NumPy is used or replicated in **Pandas**. Data in **Pandas** is often used to feed statistical analysis in SciPy, plotting functions from Matplotlib, and machine learning algorithms in Scikit-learn. |
||||
|
||||
Most of the topics we will cover today are explained and describes with examples in the first resource. The number of exercises is low on purpose: Take the time to understand the chapter 5 of the resource, even if there are 40 pages. |
||||
|
||||
## Exercises of the day |
||||
|
||||
- Exercise 1 Your first DataFrame |
||||
- Exercise 2 Electric power consumption |
||||
- Exercise 3 E-commerce purchases |
||||
- Exercise 4 Handling missing values |
||||
|
||||
## Virtual Environment |
||||
- Python 3.x |
||||
- NumPy |
||||
- Pandas |
||||
- Jupyter or JupyterLab |
||||
|
||||
*Version of Pandas I used to do the exercises: 1.0.1*. |
||||
I suggest to use the most recent one. |
||||
|
||||
## Resources |
||||
|
||||
- If I had to give you one resource it would be this one: |
||||
|
||||
https://bedford-computing.co.uk/learning/wp-content/uploads/2015/10/Python-for-Data-Analysis.pdf |
||||
|
||||
It contains ALL you need to know about Pandas. |
||||
|
||||
- Pandas documentation: |
||||
|
||||
- https://pandas.pydata.org/docs/ |
||||
|
||||
- https://jakevdp.github.io/PythonDataScienceHandbook/ |
||||
|
||||
- https://pandas.pydata.org/Pandas_Cheat_Sheet.pdf |
||||
|
||||
- https://www.learndatasci.com/tutorials/python-pandas-tutorial-complete-introduction-for-beginners/ |
||||
|
||||
- https://jakevdp.github.io/PythonDataScienceHandbook/03.04-missing-values.html |
@ -1,9 +0,0 @@
|
||||
##### The exercice is validated is all questions of the exercice are validated |
||||
|
||||
##### Activate the virtual environment. If you used `conda` run `conda activate your_env` |
||||
|
||||
##### Run `python --version` |
||||
|
||||
###### Does it print `Python 3.x`? x >= 8 |
||||
|
||||
##### Does `import jupyter`, `import numpy`, `import pandas`, `matplotlib` and `plotly` run without any error ? |
@ -1,62 +0,0 @@
|
||||
# W1D03 Piscine AI - Data Science |
||||
|
||||
## Visualizations |
||||
|
||||
While working on a dataset it is important to check the distribution of the data. Obviously, for most of humans it is difficult to visualize the data in more than 3 dimensions |
||||
|
||||
"Viz" is important to understand the data and to show results. We'll discover three libraries to visualize data in Python. These are one of the most used visualisation "libraries" in Python: |
||||
|
||||
- Pandas visualization module |
||||
- Matplotlib |
||||
- Plotly |
||||
|
||||
The goal is to understand the basics of those libraries. You'll have time during the project to master one (or the three) of them. |
||||
You may wonder why using one library is not enough. The reason is simple: it depends on the usage. |
||||
For example if you want to check the data quickly you may want to use Pandas viz module or Matplotlib. |
||||
If you want to plot a custom and more elaborated plot I suggest to use Matplotlib or Plotly. |
||||
And, if you want to create a very nice and interactive plot I suggest to use Plotly. |
||||
|
||||
|
||||
## Exercises of the day |
||||
|
||||
- Exercise 1 Pandas plot 1 |
||||
- Exercise 2 Pandas plot 2 |
||||
- Exercise 3 Matplotlib 1 |
||||
- Exercise 4 Matplotlib 2 |
||||
- Exercise 5 Matplotlib subplots |
||||
- Exercise 6 Plotly 1 |
||||
- Exercise 7 Plotly Box plots |
||||
|
||||
|
||||
## Virtual Environment |
||||
- Python 3.x |
||||
- NumPy |
||||
- Pandas |
||||
- Matplotlib |
||||
- Plotly |
||||
- Jupyter or JupyterLab |
||||
|
||||
I suggest to use the most recent version of the packages. |
||||
|
||||
## Resources |
||||
|
||||
- https://matplotlib.org/3.3.3/tutorials/index.html |
||||
- https://towardsdatascience.com/matplotlib-tutorial-learn-basics-of-pythons-powerful-plotting-library-b5d1b8f67596 |
||||
|
||||
- https://github.com/rougier/matplotlib-tutorial |
||||
- https://jakevdp.github.io/PythonDataScienceHandbook/05.13-kernel-density-estimation.html |
||||
|
||||
|
||||
# Exercise 0 Environment and libraries |
||||
|
||||
The goal of this exercise is to set up the Python work environment with the required libraries. |
||||
|
||||
**Note:** For each quest, your first exercice will be to set up the virtual environment with the required libraries. |
||||
|
||||
I recommend to use: |
||||
|
||||
- the **last stable versions** of Python. |
||||
- the virtual environment you're the most confortable with. `virtualenv` and `conda` are the most used in Data Science. |
||||
- one of the most recents versions of the libraries required |
||||
|
||||
1. Create a virtual environment named `ex00`, with a version of Python >= `3.8`, with the following libraries: `pandas`, `numpy`, `jupyter`, `matplotlib` and `plotly`. |
@ -1,8 +0,0 @@
|
||||
##### The solution of question 1 is accepted if the plot reproduces the plot in the image and respect those criteria. |
||||
|
||||
###### Does it have a the title ? |
||||
###### Does it have a name on x-axis ? |
||||
###### Does it have a legend ? |
||||
![alt text][logo] |
||||
|
||||
[logo]: ../w1day03_ex1_plot1.png "Bar plot ex1" |
@ -1,28 +0,0 @@
|
||||
# Exercise 1 Pandas plot 1 |
||||
|
||||
The goal of this exercise is to learn to create plots with use Pandas. Panda's `.plot()` is a wrapper for `matplotlib.pyplot.plot()`. |
||||
|
||||
Here is the data we will be using: |
||||
|
||||
```python |
||||
df = pd.DataFrame({ |
||||
'name':['christopher','marion','maria','mia','clement','randy','remi'], |
||||
'age':[70,30,22,19,45,33,20], |
||||
'gender':['M','F','F','F','M','M','M'], |
||||
'state':['california','dc','california','dc','california','new york','porto'], |
||||
'num_children':[2,0,0,3,8,1,4], |
||||
'num_pets':[5,1,0,5,2,2,3] |
||||
}) |
||||
``` |
||||
|
||||
1. Reproduce this plot. This plot is called a bar plot |
||||
|
||||
![alt text][logo] |
||||
|
||||
[logo]: ./w1day03_ex1_plot1.png "Bar plot ex1" |
||||
|
||||
The plot has to contain: |
||||
|
||||
- the title |
||||
- name on x-axis |
||||
- legend |
Before Width: | Height: | Size: 9.5 KiB |
@ -1,8 +0,0 @@
|
||||
##### The solution of question 1 is accepted if the plot reproduces the plot in the image and respect the following criteria. You should also observe that the older people are, the the more children they have. |
||||
|
||||
###### Does it have a the title ? |
||||
###### Does it have a name on x-axis and y-axis ? |
||||
|
||||
![alt text][logo_ex2] |
||||
|
||||
[logo_ex2]: ../w1day03_ex2_plot1.png "Scatter plot ex2" |
@ -1,26 +0,0 @@
|
||||
## Exercise 2: Pandas plot 2 |
||||
|
||||
The goal of this exercise is to learn to create plots with use Pandas. Panda's `.plot()` is a wrapper for `matplotlib.pyplot.plot()`. |
||||
|
||||
```python |
||||
df = pd.DataFrame({ |
||||
'name':['christopher','marion','maria','mia','clement','randy','remi'], |
||||
'age':[70,30,22,19,45,33,20], |
||||
'gender':['M','F','F','F','M','M','M'], |
||||
'state':['california','dc','california','dc','california','new york','porto'], |
||||
'num_children':[4,2,1,0,3,1,0], |
||||
'num_pets':[5,1,0,2,2,2,3] |
||||
}) |
||||
``` |
||||
|
||||
1. Reproduce this plot. This plot is called a scatter plot. Do you observe a relationship between the age and the number of children ? |
||||
|
||||
![alt text][logo_ex2] |
||||
|
||||
[logo_ex2]: ./w1day03_ex2_plot1.png "Scatter plot ex2" |
||||
|
||||
The plot has to contain: |
||||
|
||||
- the title |
||||
- name on x-axis |
||||
- name on y-axis |
Before Width: | Height: | Size: 11 KiB |
@ -1,11 +0,0 @@
|
||||
##### The solution of question 1 is accepted if the plot reproduces the plot in the image and respect those criteria. |
||||
|
||||
###### Does it have a the title ? |
||||
###### Does it have a name on x-axis and y-axis ? |
||||
###### Are the x-axis and y-axis limited to [1,8] ? |
||||
###### Is the line a red dashdot line with a width of 3 ? |
||||
###### Are the circles blue circles with a size of 12 ? |
||||
|
||||
![alt text][logo_ex3] |
||||
|
||||
[logo_ex3]: ../w1day03_ex3_plot1.png "Scatter plot ex3" |
@ -1,18 +0,0 @@
|
||||
## Exercise 3 Matplotlib 1 |
||||
|
||||
The goal of this plot is to learn to use Matplotlib to plot data. As you know, Matplotlib is the underlying library used by Pandas. It provides more options to plot custom visualizations. Howerver, most of the plots we will create with Matplotlib can be reproduced with Pandas' `.plot()`. |
||||
|
||||
1. Reproduce this plot. We assume the data points have integers coordinates. |
||||
|
||||
![alt text][logo_ex3] |
||||
|
||||
[logo_ex3]: ./w1day03_ex3_plot1.png "Scatter plot ex3" |
||||
|
||||
The plot has to contain: |
||||
|
||||
- the title |
||||
- name on x-axis and y-axis |
||||
- x-axis and y-axis are limited to [1,8] |
||||
- **style**: |
||||
- red dashdot line with a width of 3 |
||||
- blue circles with a size of 12 |
Before Width: | Height: | Size: 27 KiB |
@ -1,12 +0,0 @@
|
||||
##### The solution of question 1 is accepted if the plot reproduces the plot in the image and respect those criteria. |
||||
|
||||
###### Does it have a the title ? |
||||
###### Does it have a name on x-axis and y-axis ? |
||||
###### Is the left data black ? |
||||
###### Is the right data red ? |
||||
|
||||
![alt text][logo_ex4] |
||||
|
||||
[logo_ex4]: ../w1day03_ex4_plot1.png "Twin axis ex4" |
||||
|
||||
https://matplotlib.org/gallery/api/two_scales.html |
@ -1,25 +0,0 @@
|
||||
# Exercise 4 Matplotlib 2 |
||||
|
||||
The goal of this plot is to learn to use Matplotlib to plot different lines in the same plot on different axis using `twinx`. This very useful to compare variables in different ranges. |
||||
|
||||
Here is the data: |
||||
|
||||
```python |
||||
left_data = [5, 7, 11, 13, 17] |
||||
right_data = [0.1, 0.2, 0.4, 0.8, -1.6] |
||||
x_axis = [0.0, 1.0, 2.0, 3.0, 4.0] |
||||
``` |
||||
|
||||
1. Reproduce this plot |
||||
|
||||
![alt text][logo_ex4] |
||||
|
||||
[logo_ex4]: ./w1day03_ex4_plot1.png "Twin axis plot ex4" |
||||
|
||||
The plot has to contain: |
||||
|
||||
- the title |
||||
- name on left y-axis and right y-axis |
||||
- **style**: |
||||
- left data in black |
||||
- right data in red |
Before Width: | Height: | Size: 18 KiB |
@ -1,11 +0,0 @@
|
||||
##### The solution of question 1 is accepted if the plot reproduces the plot in the image and respect those criteria. |
||||
|
||||
###### Does it contain 6 subplots (2 rows, 3 columns)? |
||||
###### Does it have space between plots (`hspace=0.5` and `wspace=0.5`)? |
||||
###### Do all subplots contain a title: `Title i` ? |
||||
###### Do all subplots contain a text `(2,3,i)` centered at `(0.5, 0.5)`? *Hint*: check the parameter `ha` of `text` |
||||
###### Have all subplots been created in a for loop ? |
||||
|
||||
![alt text][logo_ex5] |
||||
|
||||
[logo_ex5]: ../w1day03_ex5_plot1.png "Subplots ex5" |
@ -1,18 +0,0 @@
|
||||
# Exercise 5 Matplotlib subplots |
||||
|
||||
The goal of this exercise is to learn to use Matplotlib to create subplots. |
||||
|
||||
1. Reproduce this plot using a **for loop**: |
||||
|
||||
![alt text][logo_ex5] |
||||
|
||||
[logo_ex5]: ./w1day03_ex5_plot1.png "Subplots ex5" |
||||
|
||||
The plot has to contain: |
||||
|
||||
- 6 subplots: 2 rows, 3 columns |
||||
- Keep space between plots: `hspace=0.5` and `wspace=0.5` |
||||
- Each plot contains |
||||
|
||||
- Text (2,3,i) centered at 0.5, 0.5. *Hint*: check the parameter `ha` of `text` |
||||
- a title: Title i |
Before Width: | Height: | Size: 13 KiB |
@ -1,25 +0,0 @@
|
||||
##### The exercice is validated is all questions of the exercice are validated |
||||
|
||||
##### The solution of question 1 is accepted if the plot reproduces the plot in the image and respect those criteria. |
||||
|
||||
###### Does it have a the title ? |
||||
###### Does it have a name on x-axis and y-axis ? |
||||
|
||||
|
||||
![alt text][logo_ex6] |
||||
|
||||
[logo_ex6]: ../w1day03_ex6_plot1.png "Time series ex6" |
||||
|
||||
|
||||
##### The solution of question 2 is accepted if the plot reproduces the plot in the image by using `plotly.graph_objects` and respect those criteria. |
||||
|
||||
|
||||
|
||||
2.This question is validated if the plot is in the image is reproduced using `plotly.graph_objects` given those criteria: |
||||
|
||||
###### Does it have a the title ? |
||||
###### Does it have a name on x-axis and y-axis ? |
||||
|
||||
![alt text][logo_ex6] |
||||
|
||||
[logo_ex6]: ../w1day03_ex6_plot1.png "Time series ex6" |
@ -1,34 +0,0 @@
|
||||
# Exercise 6 Plotly 1 |
||||
|
||||
Plotly has evolved a lot in the previous years. It is important to **always check the documentation**. |
||||
|
||||
Plotly comes with a high level interface: Plotly Express. It helps building some complex plots easily. The lesson won't detail the complex examples. Plotly express is quite interesting while using Pandas Dataframes because there are some built-in functions that leverage Pandas Dataframes. |
||||
|
||||
The plot outputed by Plotly is interactive and can also be dynamic. |
||||
|
||||
The goal of the exercise is to plot the price of a company. Its price is generated below. |
||||
|
||||
```python |
||||
returns = np.random.randn(50) |
||||
price = 100 + np.cumsum(returns) |
||||
|
||||
dates = pd.date_range(start='2020-09-01', periods=50, freq='B') |
||||
df = pd.DataFrame(zip(dates, price), |
||||
columns=['Date','Company_A']) |
||||
``` |
||||
|
||||
1. Using **Plotly express**, reproduce the plot in the image. As the data is generated randomly I do not expect you to reproduce the same line. |
||||
|
||||
![alt text][logo_ex6] |
||||
|
||||
[logo_ex6]: ./w1day03_ex6_plot1.png "Time series ex6" |
||||
|
||||
The plot has to contain: |
||||
|
||||
- title |
||||
- x-axis name |
||||
- yaxis name |
||||
|
||||
2. Same question but now using `plotly.graph_objects`. You may need to use `init_notebook_mode` from `plotly.offline`. |
||||
|
||||
https://plotly.com/python/time-series/e |
Before Width: | Height: | Size: 43 KiB |
@ -1,25 +0,0 @@
|
||||
##### The solution of question 1 is accepted if the plot reproduces the plot in the image and respect those criteria. The code below shows a solution. |
||||
|
||||
###### Does it have a the title ? |
||||
###### Does it have a legend ? |
||||
|
||||
![alt text][logo_ex7] |
||||
|
||||
[logo_ex7]: ../w1day03_ex7_plot1.png "Box plot ex7" |
||||
|
||||
```python |
||||
import plotly.graph_objects as go |
||||
import numpy as np |
||||
|
||||
y0 = np.random.randn(50) |
||||
y1 = np.random.randn(50) + 1 # shift mean |
||||
y2 = np.random.randn(50) + 2 |
||||
|
||||
fig = go.Figure() |
||||
fig.add_trace(go.Box(y=y0, name='Sample A', |
||||
marker_color = 'indianred')) |
||||
fig.add_trace(go.Box(y=y1, name = 'Sample B', |
||||
marker_color = 'lightseagreen')) |
||||
|
||||
fig.show() |
||||
``` |
@ -1,24 +0,0 @@
|
||||
# Exercise 7 Plotly Box plots |
||||
|
||||
The goal of this exercise is to learn to use Plotly to plot Box Plots. It is t is a method for graphically depicting groups of numerical data through their quartiles and values as min, max. It allows to compare quickly some variables. |
||||
|
||||
Let us generate 3 random arrays from a normal distribution. And for each array add respectively 1, 2 to the normal distribution. |
||||
|
||||
```python |
||||
y0 = np.random.randn(50) |
||||
y1 = np.random.randn(50) + 1 # shift mean |
||||
y2 = np.random.randn(50) + 2 |
||||
``` |
||||
|
||||
1. Plot in the same Figure 2 box plots as shown in the image. In this exercise the style is not important. |
||||
|
||||
![alt text][logo_ex7] |
||||
|
||||
[logo_ex7]: ./w1day03_ex7_plot1.png "Box plot ex7" |
||||
|
||||
The plot has to contain: |
||||
|
||||
- the title |
||||
- the legend |
||||
|
||||
https://plotly.com/python/box-plots/ |
Before Width: | Height: | Size: 13 KiB |
@ -1,47 +0,0 @@
|
||||
# W1D03 Piscine AI - Data Science |
||||
|
||||
## Visualizations |
||||
|
||||
While working on a dataset it is important to check the distribution of the data. Obviously, for most of humans it is difficult to visualize the data in more than 3 dimensions |
||||
|
||||
"Viz" is important to understand the data and to show results. We'll discover three libraries to visualize data in Python. These are one of the most used visualisation "libraries" in Python: |
||||
|
||||
- Pandas visualization module |
||||
- Matplotlib |
||||
- Plotly |
||||
|
||||
The goal is to understand the basics of those libraries. You'll have time during the project to master one (or the three) of them. |
||||
You may wonder why using one library is not enough. The reason is simple: it depends on the usage. |
||||
For example if you want to check the data quickly you may want to use Pandas viz module or Matplotlib. |
||||
If you want to plot a custom and more elaborated plot I suggest to use Matplotlib or Plotly. |
||||
And, if you want to create a very nice and interactive plot I suggest to use Plotly. |
||||
|
||||
|
||||
## Exercises of the day |
||||
|
||||
- Exercise 1 Pandas plot 1 |
||||
- Exercise 2 Pandas plot 2 |
||||
- Exercise 3 Matplotlib 1 |
||||
- Exercise 4 Matplotlib 2 |
||||
- Exercise 5 Matplotlib subplots |
||||
- Exercise 6 Plotly 1 |
||||
- Exercise 7 Plotly Box plots |
||||
|
||||
|
||||
## Virtual Environment |
||||
- Python 3.x |
||||
- NumPy |
||||
- Pandas |
||||
- Matplotlib |
||||
- Plotly |
||||
- Jupyter or JupyterLab |
||||
|
||||
I suggest to use the most recent version of the packages. |
||||
|
||||
## Resources |
||||
|
||||
- https://matplotlib.org/3.3.3/tutorials/index.html |
||||
- https://towardsdatascience.com/matplotlib-tutorial-learn-basics-of-pythons-powerful-plotting-library-b5d1b8f67596 |
||||
|
||||
- https://github.com/rougier/matplotlib-tutorial |
||||
- https://jakevdp.github.io/PythonDataScienceHandbook/05.13-kernel-density-estimation.html |
@ -1,9 +0,0 @@
|
||||
##### The exercice is validated is all questions of the exercice are validated |
||||
|
||||
##### Activate the virtual environment. If you used `conda` run `conda activate your_env` |
||||
|
||||
##### Run `python --version` |
||||
|
||||
###### Does it print `Python 3.x`? x >= 8 |
||||
|
||||
##### Does `import jupyter`, `import numpy` and `import pandas` run without any error ? |
@ -1,55 +0,0 @@
|
||||
# W1D04 Piscine AI - Data Science |
||||
|
||||
## Data wrangling with Pandas |
||||
|
||||
Data wrangling is one of the crucial tasks in data science and analysis which includes operations like: |
||||
|
||||
- Data Sorting: To rearrange values in ascending or descending order. |
||||
- Data Filtration: To create a subset of available data. |
||||
- Data Reduction: To eliminate or replace unwanted values. |
||||
- Data Access: To read or write data files. |
||||
- Data Processing: To perform aggregation, statistical, and similar operations on specific values. |
||||
Ax explained before, Pandas is an open source library, specifically developed for data science and analysis. It is built upon the Numpy (to handle numeric data in tabular form) package and has inbuilt data structures to ease-up the process of data manipulation, aka data munging/wrangling. |
||||
|
||||
## Exercises of the day |
||||
|
||||
- Exercise 1 Concatenate |
||||
- Exercise 2 Merge |
||||
- Exercise 3 Merge MultiIndex |
||||
- Exercise 4 Groupby Apply |
||||
- Exercise 5 Groupby Agg |
||||
- Exercise 6 Unstack |
||||
|
||||
## Virtual Environment |
||||
- Python 3.x |
||||
- NumPy |
||||
- Pandas |
||||
- Jupyter or JupyterLab |
||||
|
||||
*Version of Pandas I used to do the exercises: 1.0.1*. |
||||
I suggest to use the most recent one. |
||||
|
||||
## Resources |
||||
|
||||
- https://jakevdp.github.io/PythonDataScienceHandbook/ |
||||
|
||||
- https://pandas.pydata.org/Pandas_Cheat_Sheet.pdf |
||||
|
||||
- https://www.learndatasci.com/tutorials/python-pandas-tutorial-complete-introduction-for-beginners/ |
||||
|
||||
- https://towardsdatascience.com/different-ways-to-iterate-over-rows-in-a-pandas-dataframe-performance-comparison-dc0d5dcef8fe |
||||
|
||||
|
||||
# Exercise 0 Environment and libraries |
||||
|
||||
The goal of this exercise is to set up the Python work environment with the required libraries. |
||||
|
||||
**Note:** For each quest, your first exercice will be to set up the virtual environment with the required libraries. |
||||
|
||||
I recommend to use: |
||||
|
||||
- the **last stable versions** of Python. |
||||
- the virtual environment you're the most confortable with. `virtualenv` and `conda` are the most used in Data Science. |
||||
- one of the most recents versions of the libraries required |
||||
|
||||
1. Create a virtual environment named `ex00`, with a version of Python >= `3.8`, with the following libraries: `pandas`, `numpy` and `jupyter`. |
@ -1,8 +0,0 @@
|
||||
##### This question is validated if the outputted DataFrame is: |
||||
|
||||
| | letter | number | |
||||
|---:|:---------|---------:| |
||||
| 0 | a | 1 | |
||||
| 1 | b | 2 | |
||||
| 2 | c | 1 | |
||||
| 3 | d | 2 | |
@ -1,14 +0,0 @@
|
||||
# Exercise 1 Concatenate |
||||
|
||||
The goal of this exercise is to learn to concatenate DataFrames. The logic is the same for the Series. |
||||
|
||||
Here are the two DataFrames to concatenate: |
||||
|
||||
```python |
||||
df1 = pd.DataFrame([['a', 1], ['b', 2]], |
||||
columns=['letter', 'number']) |
||||
df2 = pd.DataFrame([['c', 1], ['d', 2]], |
||||
columns=['letter', 'number']) |
||||
``` |
||||
|
||||
1. Concatenate this two DataFrames on index axis and reset the index. The index of the outputted should be `RangeIndex(start=0, stop=4, step=1)`. **Do not change the index manually**. |
@ -1,23 +0,0 @@
|
||||
##### The exercice is validated is all questions of the exercice are validated |
||||
|
||||
##### The question 1 is validated if the output is: |
||||
|
||||
| | id | Feature1_x | Feature2_x | Feature1_y | Feature2_y | |
||||
|---:|-----:|:-------------|:-------------|:-------------|:-------------| |
||||
| 0 | 1 | A | B | K | L | |
||||
| 1 | 2 | C | D | M | N | |
||||
|
||||
##### The question 2 is validated if the output is: |
||||
|
||||
| | id | Feature1_df1 | Feature2_df1 | Feature1_df2 | Feature2_df2 | |
||||
|---:|-----:|:---------------|:---------------|:---------------|:---------------| |
||||
| 0 | 1 | A | B | K | L | |
||||
| 1 | 2 | C | D | M | N | |
||||
| 2 | 3 | E | F | nan | nan | |
||||
| 3 | 4 | G | H | nan | nan | |
||||
| 4 | 5 | I | J | nan | nan | |
||||
| 5 | 6 | nan | nan | O | P | |
||||
| 6 | 7 | nan | nan | Q | R | |
||||
| 7 | 8 | nan | nan | S | T | |
||||
|
||||
Note: Check that the suffixes are set using the suffix parameters rather than manually changing the columns' name. |
@ -1,46 +0,0 @@
|
||||
# Exercise 2 Merge |
||||
|
||||
The goal of this exercise is to learn to merge DataFrames |
||||
The logic of merging DataFrames in Pandas is quite similar as the one used in SQL. |
||||
|
||||
Here are the two DataFrames to merge: |
||||
|
||||
```python |
||||
#df1 |
||||
|
||||
df1_dict = { |
||||
'id': ['1', '2', '3', '4', '5'], |
||||
'Feature1': ['A', 'C', 'E', 'G', 'I'], |
||||
'Feature2': ['B', 'D', 'F', 'H', 'J']} |
||||
|
||||
df1 = pd.DataFrame(df1_dict, columns = ['id', 'Feature1', 'Feature2']) |
||||
|
||||
#df2 |
||||
df2_dict = { |
||||
'id': ['1', '2', '6', '7', '8'], |
||||
'Feature1': ['K', 'M', 'O', 'Q', 'S'], |
||||
'Feature2': ['L', 'N', 'P', 'R', 'T']} |
||||
|
||||
df2 = pd.DataFrame(df2_dict, columns = ['id', 'Feature1', 'Feature2']) |
||||
``` |
||||
|
||||
1. Merge the two DataFrames to get this output: |
||||
|
||||
| | id | Feature1_x | Feature2_x | Feature1_y | Feature2_y | |
||||
|---:|-----:|:-------------|:-------------|:-------------|:-------------| |
||||
| 0 | 1 | A | B | K | L | |
||||
| 1 | 2 | C | D | M | N | |
||||
|
||||
2. Merge the two DataFrames to get this output: |
||||
|
||||
| | id | Feature1_df1 | Feature2_df1 | Feature1_df2 | Feature2_df2 | |
||||
|---:|-----:|:---------------|:---------------|:---------------|:---------------| |
||||
| 0 | 1 | A | B | K | L | |
||||
| 1 | 2 | C | D | M | N | |
||||
| 2 | 3 | E | F | nan | nan | |
||||
| 3 | 4 | G | H | nan | nan | |
||||
| 4 | 5 | I | J | nan | nan | |
||||
| 5 | 6 | nan | nan | O | P | |
||||
| 6 | 7 | nan | nan | Q | R | |
||||
| 7 | 8 | nan | nan | S | T | |
||||
|
@ -1,14 +0,0 @@
|
||||
##### The exercice is validated is all questions of the exercice are validated |
||||
|
||||
##### The question 1 is validated if the outputted DataFrame's shape is `(1305, 5)` and if `merged.head()` returns a table as below. One of the answers that returns the correct DataFrame is `market_data.merge(alternative_data, how='left', left_index=True, right_index=True)` |
||||
|
||||
| | Open | Close | Close_Adjusted | Twitter | Reddit | |
||||
|:-----------------------------------------------------|-----------:|----------:|-----------------:|------------:|----------:| |
||||
| (Timestamp('2021-01-01 00:00:00', freq='B'), 'AAPL') | 0.0991792 | -0.31603 | 0.634787 | -0.00159041 | 1.06053 | |
||||
| (Timestamp('2021-01-01 00:00:00', freq='B'), 'FB') | -0.123753 | 1.00269 | 0.713264 | 0.0142127 | -0.487028 | |
||||
| (Timestamp('2021-01-01 00:00:00', freq='B'), 'GE') | -1.37775 | -1.01504 | 1.2858 | 0.109835 | 0.04273 | |
||||
| (Timestamp('2021-01-01 00:00:00', freq='B'), 'AMZN') | 1.06324 | 0.841241 | -0.799481 | -0.805677 | 0.511769 | |
||||
| (Timestamp('2021-01-01 00:00:00', freq='B'), 'DAI') | -0.603453 | -2.06141 | -0.969064 | 1.49817 | 0.730055 | |
||||
|
||||
|
||||
##### The question 2 is validated if the numbers that are missing in the DataFrame are equal to 0 and if `filled_df.sum().sum() == merged_df.sum().sum()` gives: `True` |
@ -1,34 +0,0 @@
|
||||
# Exercise 3 Merge MultiIndex |
||||
|
||||
The goal of this exercise is to learn to merge DataFrames with MultiIndex. |
||||
Use the code below to generate the DataFrames. `market_data` contains fake market data. In finance, the market is available during the trading days (business days). `alternative_data` contains fake alternative data from social media. This data is available every day. But, for some reasons the Data Engineer lost the last 15 days of alternative data. |
||||
|
||||
1. Using `market_data` as the reference, merge `alternative_data` on `market_data` |
||||
|
||||
```python |
||||
#generate days |
||||
all_dates = pd.date_range('2021-01-01', '2021-12-15') |
||||
business_dates = pd.bdate_range('2021-01-01', '2021-12-31') |
||||
|
||||
#generate tickers |
||||
tickers = ['AAPL', 'FB', 'GE', 'AMZN', 'DAI'] |
||||
|
||||
#create indexs |
||||
index_alt = pd.MultiIndex.from_product([all_dates, tickers], names=['Date', 'Ticker']) |
||||
index = pd.MultiIndex.from_product([business_dates, tickers], names=['Date', 'Ticker']) |
||||
|
||||
# create DFs |
||||
market_data = pd.DataFrame(index=index, |
||||
data=np.random.randn(len(index), 3), |
||||
columns=['Open','Close','Close_Adjusted']) |
||||
|
||||
alternative_data = pd.DataFrame(index=index_alt, |
||||
data=np.random.randn(len(index_alt), 2), |
||||
columns=['Twitter','Reddit']) |
||||
``` |
||||
|
||||
`reset_index` is not allowed for this question |
||||
|
||||
2. Fill missing values with 0 |
||||
|
||||
- https://medium.com/swlh/merging-dataframes-with-pandas-pd-merge-7764c7e2d46d |
@ -1,56 +0,0 @@
|
||||
##### The exercice is validated is all questions of the exercice are validated and if the for loop hasn't been used. The goal is to use `groupby` and `apply`. |
||||
|
||||
##### The question 1 is validated if the output is: |
||||
|
||||
```python |
||||
df = pd.DataFrame(range(1,11), columns=['sequence']) |
||||
print(winsorize(df, [0.20, 0.80]).to_markdown()) |
||||
``` |
||||
|
||||
| | sequence | |
||||
|---:|-----------:| |
||||
| 0 | 2.8 | |
||||
| 1 | 2.8 | |
||||
| 2 | 3 | |
||||
| 3 | 4 | |
||||
| 4 | 5 | |
||||
| 5 | 6 | |
||||
| 6 | 7 | |
||||
| 7 | 8 | |
||||
| 8 | 8.2 | |
||||
| 9 | 8.2 | |
||||
|
||||
##### The question 2 is validated if the output is a Pandas Series or DataFrame with the first 11 rows equal to the output below. The code below give a solution. |
||||
|
||||
| | sequence | |
||||
|---:|-----------:| |
||||
| 0 | 1.45 | |
||||
| 1 | 2 | |
||||
| 2 | 3 | |
||||
| 3 | 4 | |
||||
| 4 | 5 | |
||||
| 5 | 6 | |
||||
| 6 | 7 | |
||||
| 7 | 8 | |
||||
| 8 | 9 | |
||||
| 9 | 9.55 | |
||||
| 10 | 11.45 | |
||||
|
||||
|
||||
```python |
||||
def winsorize(df_series, quantiles): |
||||
""" |
||||
df: pd.DataFrame or pd.Series |
||||
quantiles: list [0.05, 0.95] |
||||
|
||||
""" |
||||
min_value = np.quantile(df_series, quantiles[0]) |
||||
max_value = np.quantile(df_series, quantiles[1]) |
||||
|
||||
return df_series.clip(lower = min_value, upper = max_value) |
||||
|
||||
|
||||
df.groupby("group")[['sequence']].apply(winsorize, [0.05,0.95]) |
||||
``` |
||||
|
||||
- https://towardsdatascience.com/how-to-use-the-split-apply-combine-strategy-in-pandas-groupby-29e0eb44b62e |
@ -1,65 +0,0 @@
|
||||
# Exercise 4 Groupby Apply |
||||
|
||||
The goal of this exercise is to learn to group the data and apply a function on the groups. |
||||
The use case we will work on is computing |
||||
|
||||
1. Create a function that uses `pandas.DataFrame.clip` and that replace extreme values by a given percentile. The values that are greater than the upper percentile 80% are replaced by the percentile 80%. The values that are smaller than the lower percentile 20% are replaced by the percentile 20%. This process that correct outliers is called **winsorizing**. |
||||
I recommend to use NumPy to compute the percentiles to make sure we used the same default parameters. |
||||
|
||||
```python |
||||
def winsorize(df, quantiles): |
||||
""" |
||||
df: pd.DataFrame |
||||
quantiles: list |
||||
ex: [0.05, 0.95] |
||||
""" |
||||
#TODO |
||||
return |
||||
``` |
||||
|
||||
Here is what the function should output: |
||||
|
||||
```python |
||||
df = pd.DataFrame(range(1,11), columns=['sequence']) |
||||
print(winsorize(df, [0.20, 0.80]).to_markdown()) |
||||
|
||||
``` |
||||
|
||||
| | sequence | |
||||
|---:|-----------:| |
||||
| 0 | 2.8 | |
||||
| 1 | 2.8 | |
||||
| 2 | 3 | |
||||
| 3 | 4 | |
||||
| 4 | 5 | |
||||
| 5 | 6 | |
||||
| 6 | 7 | |
||||
| 7 | 8 | |
||||
| 8 | 8.2 | |
||||
| 9 | 8.2 | |
||||
|
||||
2. Now we consider that each value belongs to a group. The goal is to apply the **winsorizing to each group**. In this question we use winsorizing values that are common: `[0.05,0.95]` as percentiles. Here is the new data set: |
||||
|
||||
```python |
||||
groups = np.concatenate([np.ones(10), np.ones(10)+1, np.ones(10)+2, np.ones(10)+3, np.ones(10)+4]) |
||||
|
||||
df = pd.DataFrame(data= zip(groups, |
||||
range(1,51)), |
||||
columns=["group", "sequence"]) |
||||
``` |
||||
|
||||
The expected output (first rows) is: |
||||
|
||||
| | sequence | |
||||
|---:|-----------:| |
||||
| 0 | 1.45 | |
||||
| 1 | 2 | |
||||
| 2 | 3 | |
||||
| 3 | 4 | |
||||
| 4 | 5 | |
||||
| 5 | 6 | |
||||
| 6 | 7 | |
||||
| 7 | 8 | |
||||
| 8 | 9 | |
||||
| 9 | 9.55 | |
||||
| 10 | 11.45 | |
@ -1,8 +0,0 @@
|
||||
##### The question is validated if the output is as below. The columns don't have to be MultiIndex. A solution could be `df.groupby('product').agg({'value':['min','max','mean']})` |
||||
|
||||
|
||||
| product | ('value', 'min') | ('value', 'max') | ('value', 'mean') | |
||||
|:-------------|-------------------:|-------------------:|--------------------:| |
||||
| chair | 22.89 | 32.12 | 27.505 | |
||||
| mobile phone | 100 | 111.22 | 105.61 | |
||||
| table | 20.45 | 99.99 | 51.22 | |
@ -1,23 +0,0 @@
|
||||
# Exercise 5 Groupby Agg |
||||
|
||||
The goal of this exercise is to learn to compute different type of aggregations on the groups. This small DataFrame contains products and prices. |
||||
|
||||
| | value | product | |
||||
|---:|--------:|:-------------| |
||||
| 0 | 20.45 | table | |
||||
| 1 | 22.89 | chair | |
||||
| 2 | 32.12 | chair | |
||||
| 3 | 111.22 | mobile phone | |
||||
| 4 | 33.22 | table | |
||||
| 5 | 100 | mobile phone | |
||||
| 6 | 99.99 | table | |
||||
|
||||
1. Compute the min, max and mean price for each product in one single line of code. The expected output is: |
||||
|
||||
| product | ('value', 'min') | ('value', 'max') | ('value', 'mean') | |
||||
|:-------------|-------------------:|-------------------:|--------------------:| |
||||
| chair | 22.89 | 32.12 | 27.505 | |
||||
| mobile phone | 100 | 111.22 | 105.61 | |
||||
| table | 20.45 | 99.99 | 51.22 | |
||||
|
||||
Note: The columns don't have to be MultiIndex |
@ -1,32 +0,0 @@
|
||||
# Exercise 6 Unstack |
||||
|
||||
The goal of this exercise is to learn to unstack a MultiIndex |
||||
Let's assume we trained a machine learning model that predicts a daily score on the companies (tickers) below. It may be very useful to unstack the MultiIndex: plot the time series, vectorize the backtest, ... |
||||
|
||||
```python |
||||
business_dates = pd.bdate_range('2021-01-01', '2021-12-31') |
||||
|
||||
#generate tickers |
||||
tickers = ['AAPL', 'FB', 'GE', 'AMZN', 'DAI'] |
||||
|
||||
#create indexs |
||||
index = pd.MultiIndex.from_product([business_dates, tickers], names=['Date', 'Ticker']) |
||||
|
||||
# create DFs |
||||
market_data = pd.DataFrame(index=index, |
||||
data=np.random.randn(len(index), 1), |
||||
columns=['Prediction']) |
||||
|
||||
``` |
||||
|
||||
1. Unstack the DataFrame. |
||||
|
||||
The first 3 rows of the DataFrame should like this: |
||||
|
||||
| Date | ('Prediction', 'AAPL') | ('Prediction', 'AMZN') | ('Prediction', 'DAI') | ('Prediction', 'FB') | ('Prediction', 'GE') | |
||||
|:--------------------|-------------------------:|-------------------------:|------------------------:|-----------------------:|-----------------------:| |
||||
| 2021-01-01 00:00:00 | 0.382312 | -0.072392 | -0.551167 | -0.0585555 | 1.05955 | |
||||
| 2021-01-04 00:00:00 | -0.560953 | 0.503199 | -0.79517 | -3.23136 | 1.50271 | |
||||
| 2021-01-05 00:00:00 | 0.211489 | 1.84867 | 0.287906 | -1.81119 | 1.20321 | |
||||
|
||||
2. Plot the 5 times series in the same plot using Pandas built-in visualization functions with a title. |
@ -1,40 +0,0 @@
|
||||
# W1D04 Piscine AI - Data Science |
||||
|
||||
## Data wrangling with Pandas |
||||
|
||||
Data wrangling is one of the crucial tasks in data science and analysis which includes operations like: |
||||
|
||||
- Data Sorting: To rearrange values in ascending or descending order. |
||||
- Data Filtration: To create a subset of available data. |
||||
- Data Reduction: To eliminate or replace unwanted values. |
||||
- Data Access: To read or write data files. |
||||
- Data Processing: To perform aggregation, statistical, and similar operations on specific values. |
||||
Ax explained before, Pandas is an open source library, specifically developed for data science and analysis. It is built upon the Numpy (to handle numeric data in tabular form) package and has inbuilt data structures to ease-up the process of data manipulation, aka data munging/wrangling. |
||||
|
||||
## Exercises of the day |
||||
|
||||
- Exercise 1 Concatenate |
||||
- Exercise 2 Merge |
||||
- Exercise 3 Merge MultiIndex |
||||
- Exercise 4 Groupby Apply |
||||
- Exercise 5 Groupby Agg |
||||
- Exercise 6 Unstack |
||||
|
||||
## Virtual Environment |
||||
- Python 3.x |
||||
- NumPy |
||||
- Pandas |
||||
- Jupyter or JupyterLab |
||||
|
||||
*Version of Pandas I used to do the exercises: 1.0.1*. |
||||
I suggest to use the most recent one. |
||||
|
||||
## Resources |
||||
|
||||
- https://jakevdp.github.io/PythonDataScienceHandbook/ |
||||
|
||||
- https://pandas.pydata.org/Pandas_Cheat_Sheet.pdf |
||||
|
||||
- https://www.learndatasci.com/tutorials/python-pandas-tutorial-complete-introduction-for-beginners/ |
||||
|
||||
- https://towardsdatascience.com/different-ways-to-iterate-over-rows-in-a-pandas-dataframe-performance-comparison-dc0d5dcef8fe |
@ -1,9 +0,0 @@
|
||||
##### The exercice is validated is all questions of the exercice are validated |
||||
|
||||
##### Activate the virtual environment. If you used `conda` run `conda activate your_env` |
||||
|
||||
##### Run `python --version` |
||||
|
||||
###### Does it print `Python 3.x`? x >= 8 |
||||
|
||||
##### Does `import jupyter`, `import numpy` and `import pandas` run without any error ? |
@ -1,52 +0,0 @@
|
||||
# W1D05 Piscine AI - Data Science |
||||
|
||||
## Time Series with Pandas |
||||
|
||||
Time series data are data that are indexed by a sequence of dates or times. Today, you'll learn how to use methods built into Pandas to work with this index. You'll also learn for instance: |
||||
- to resample time series to change the frequency |
||||
- to calculate rolling and cumulative values for times series |
||||
- to build a backtest |
||||
|
||||
Time series a used A LOT in finance. You'll learn to evaluate financial strategies using Pandas. It is important to keep in mind that Python is vectorized. That's why some questions constraint you to not use a for loop ;-). |
||||
|
||||
## Exercises of the day |
||||
|
||||
- Exercise 1 Series |
||||
- Exercise 2 Financial data |
||||
- Exercise 3 Multi asset returns |
||||
- Exercise 4 Backtest |
||||
|
||||
|
||||
## Virtual Environment |
||||
- Python 3.x |
||||
- NumPy |
||||
- Pandas |
||||
- Jupyter or JupyterLab |
||||
|
||||
*Version of Pandas I used to do the exercises: 1.0.1*. |
||||
I suggest to use the most recent one. |
||||
|
||||
## Resources |
||||
|
||||
- https://jakevdp.github.io/PythonDataScienceHandbook/ |
||||
|
||||
- https://pandas.pydata.org/Pandas_Cheat_Sheet.pdf |
||||
|
||||
- https://www.learndatasci.com/tutorials/python-pandas-tutorial-complete-introduction-for-beginners/ |
||||
|
||||
- https://towardsdatascience.com/different-ways-to-iterate-over-rows-in-a-pandas-dataframe-performance-comparison-dc0d5dcef8fe |
||||
|
||||
|
||||
# Exercise 0 Environment and libraries |
||||
|
||||
The goal of this exercise is to set up the Python work environment with the required libraries. |
||||
|
||||
**Note:** For each quest, your first exercice will be to set up the virtual environment with the required libraries. |
||||
|
||||
I recommend to use: |
||||
|
||||
- the **last stable versions** of Python. |
||||
- the virtual environment you're the most confortable with. `virtualenv` and `conda` are the most used in Data Science. |
||||
- one of the most recents versions of the libraries required |
||||
|
||||
1. Create a virtual environment named `ex00`, with a version of Python >= `3.8`, with the following libraries: `pandas`, `numpy` and `jupyter`. |
@ -1,35 +0,0 @@
|
||||
##### The exercice is validated is all questions of the exercice are validated |
||||
|
||||
##### The question 1 is validated if the output of is as below. The best solution uses `pd.date_range` to generate the index and `range` to generate the integer series. |
||||
|
||||
```console |
||||
2010-01-01 0 |
||||
2010-01-02 1 |
||||
2010-01-03 2 |
||||
2010-01-04 3 |
||||
2010-01-05 4 |
||||
... |
||||
2020-12-27 4013 |
||||
2020-12-28 4014 |
||||
2020-12-29 4015 |
||||
2020-12-30 4016 |
||||
2020-12-31 4017 |
||||
Freq: D, Name: integer_series, Length: 4018, dtype: int64 |
||||
``` |
||||
|
||||
##### This question is validated if the output is as below. If the `NaN` values have been dropped the solution is also accepted. The solution uses `rolling().mean()`. |
||||
|
||||
```console |
||||
2010-01-01 NaN |
||||
2010-01-02 NaN |
||||
2010-01-03 NaN |
||||
2010-01-04 NaN |
||||
2010-01-05 NaN |
||||
... |
||||
2020-12-27 4010.0 |
||||
2020-12-28 4011.0 |
||||
2020-12-29 4012.0 |
||||
2020-12-30 4013.0 |
||||
2020-12-31 4014.0 |
||||
Freq: D, Name: integer_series, Length: 4018, dtype: float64 |
||||
``` |
@ -1,7 +0,0 @@
|
||||
# Exercise 1 |
||||
|
||||
The goal of this exercise is to learn to manipulate time series in Pandas. |
||||
|
||||
1. Create a `Series` named `integer_series` from 1st January 2010 to 31 December 2020. At each date is associated the number of days since 1st January 2010. It starts with 0. |
||||
|
||||
2. Using Pandas, compute a 7 days moving average. This transformation smooths the time series by removing small fluctuations. **without for loop** |